SEARCH
ALL NEWS
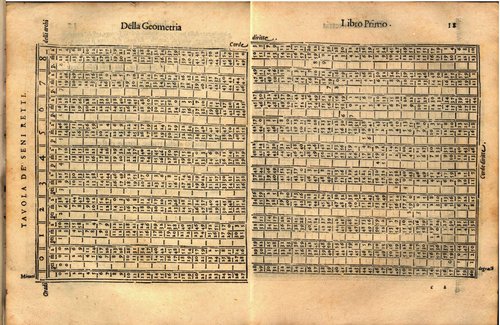
Explainable AI illuminates the course of history
Understanding the evolution and dissemination of human knowledge over time is a long-cherished dream of many historians. A dream that faced many challenges due to the abundance of historical materials and limited specialist resources. However, the digitization of many historical archives presents new opportunities for AI-supported analysis.
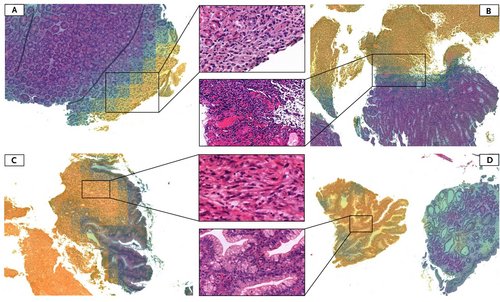
AI in medicine: new approach for more efficient diagnostics
Researchers from LMU, BIFOLD, and Charité have developed a new AI tool that uses imaging data to also detect less frequent diseases of the gastrointestinal tract. In contrast to conventional models, the new AI only needs training data from common findings to detect deviations.
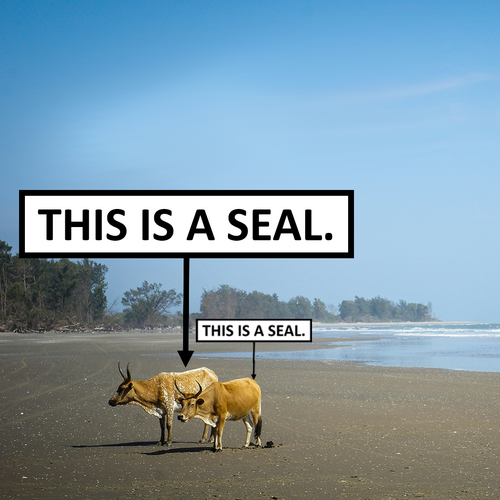
Publication Highlight – Pruning Clever-Hans strategies
Hidden Clever-Hans effects can undermine the reliability of AI models. The paper “Preemptively pruning Clever-Hans strategies in deep neural networks” introduces a method that corrects biases in neural networks without prior knowledge of faulty features.
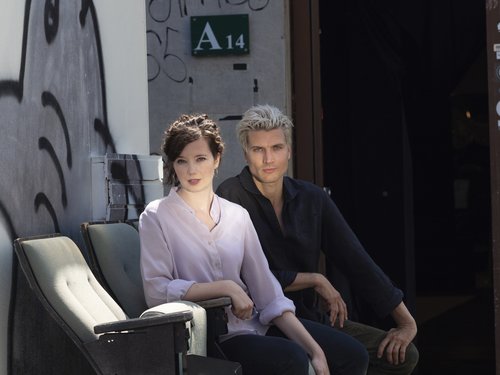
A Dialogue of Art and Science
BIFOLD is happy to announce the Berlin-based artist duo kennedy+swan as Artists in Residence 2024/2025. Until June 2025, kennedy+swan will collaborate with BIFOLD researchers as part of the “Art of Entanglement” Artist in Residence program, which aims to bridge artistic and scientific perspectives on artificial intelligence.
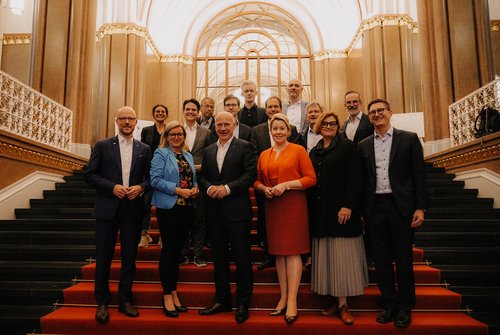
The Berlin AI Roundtable
At the invitation of the Governing Mayor of Berlin, Kai Wegner, key players from business, academia, and digitalization gathered for a cross-departmental AI roundtable at the Red Town Hall.
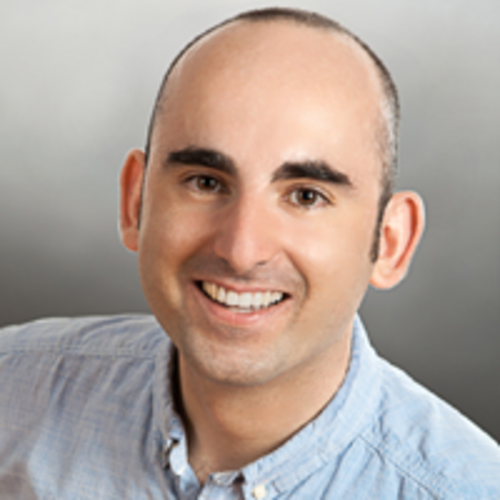
Researcher Spotlight: Dr. Viktor Rosenfeld
Dr. Viktor Rosenfeld did his PhD in the "Database Systems and Information Management" research group led by Professor Dr. Volker Markl. Learn more about Viktor's passion for database operators and singing in public.
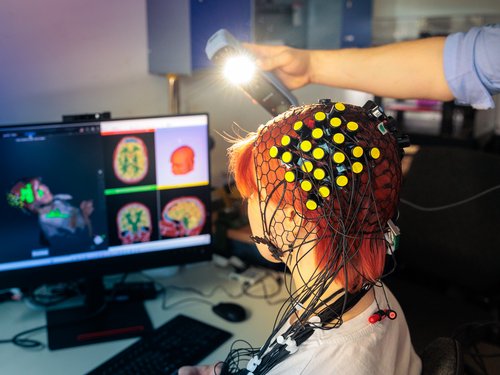
Expedition Neurotechnology
Join us for a tour around the IBS Lab: The Lab is chaired by Dr. Alexander von Lühmann, who recently received a ERC Starting Grant, for his researchproject to measure and analyze brain activity in people's everyday environments, using AI to analyze the detected signals.
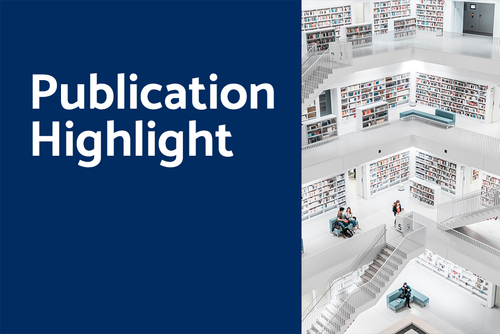
Publication Highlight - Snapcase
At the VLDB 2024 conference, the BIFOLD Research Group DEEM Lab introduced "Snapcase," a demo paper that addresses the concept of machine unlearning.
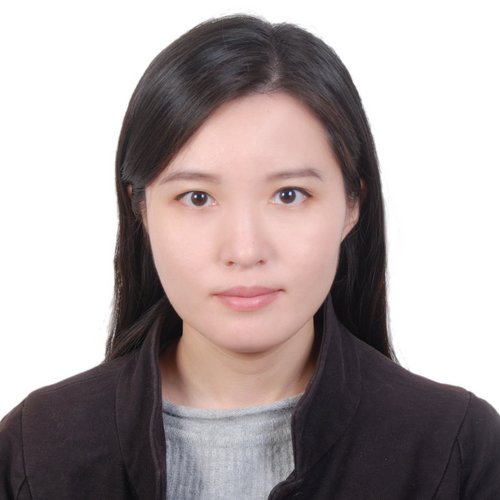
Researcher Spotlight: Dr. Binger Chen
Get to know BIFOLD researcher Dr. Binger Chen. She recently successfully completed her PhD with Summa cum Laude. Glimpse into her research utilizing Big Data and Machine Learning technology to create representations of programming language features.
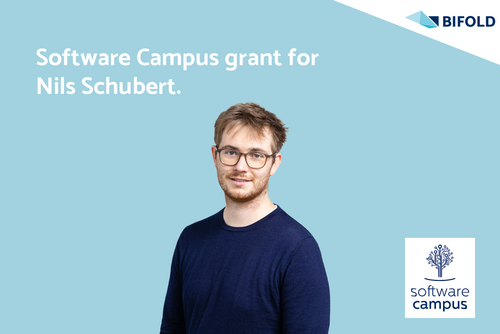
Software Campus grant for BIFOLD PhD student
BIFOLD PhD student Nils Schubert has been awarded a Software Campus 2024 grant to conduct his own research project as junior research lead.