NEWS
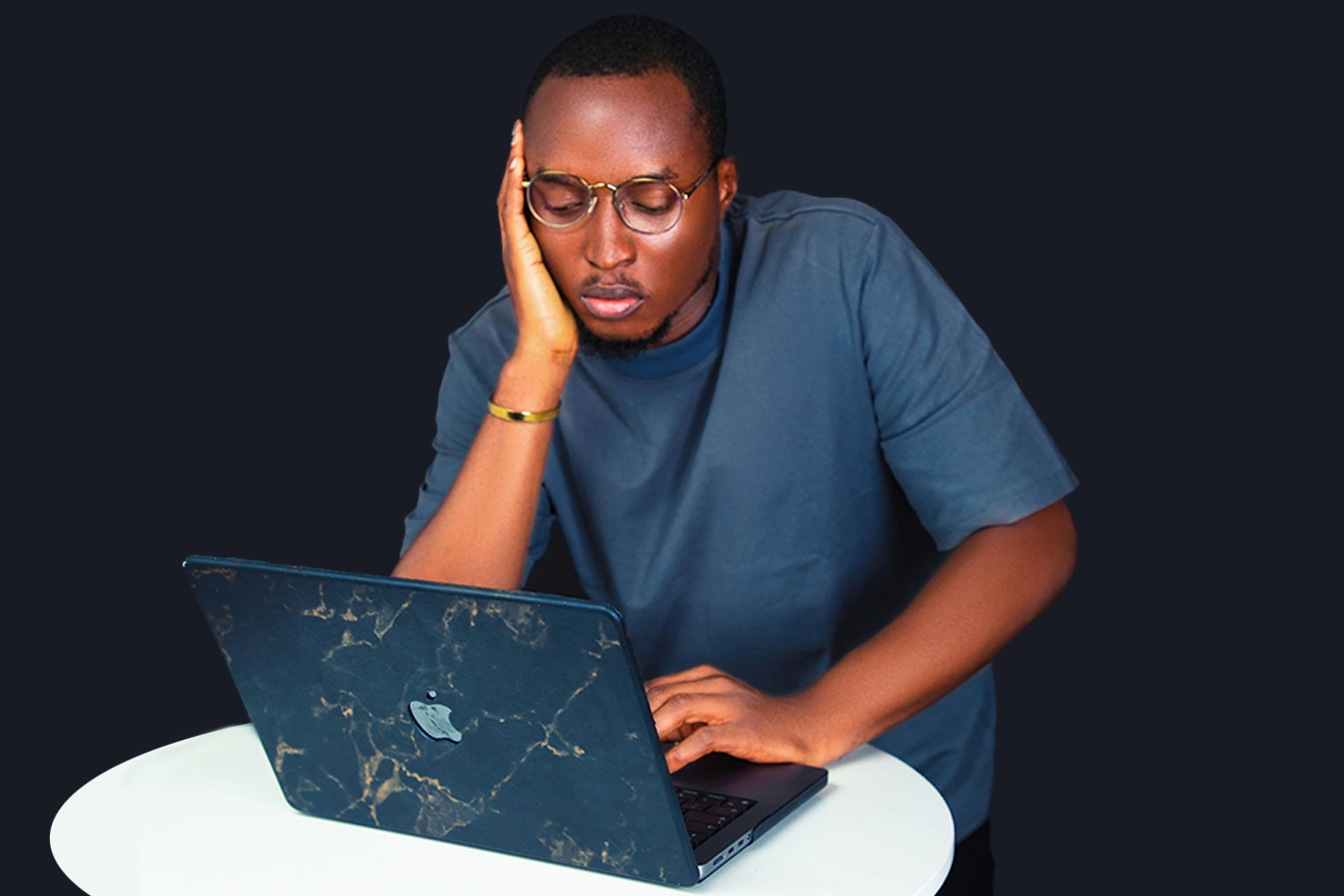
“POLAR” lowers the adoption barrier for adaptive query processing in database systems
A preprint by BIFOLD researchers titled "POLAR: Adaptive and Non-invasive Join Order Selection via Plans of Least Resistance" is set to be presented at the VLDB conference in 2024. The database engineering paper introduces a technique for reordering joins that is adaptive, with a focus on non-invasive integration and low overhead.
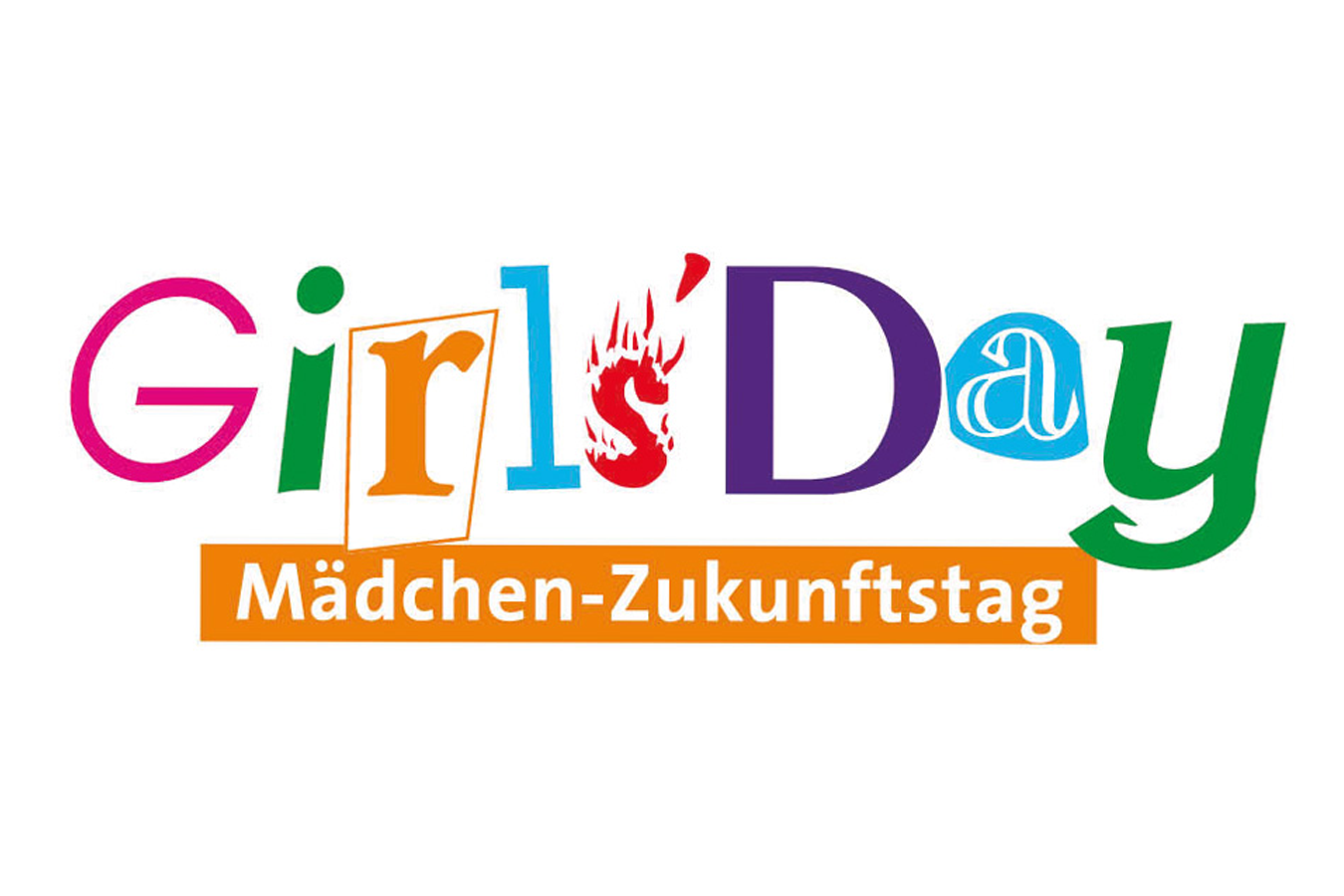
Girls' Day: dEIn Labor and BIFOLD offer AI workshop
TU Berlin is once again participating in this year's "Girls' Day" on April 25, 2024. dEIn Labor and BIFOLD offer a workshop for schoolgirls from the age of 15.
EVENTS
BIFOLD Colloquium 04/2024
Amr El Abbadi is a Computer Science Professor at the University of California, Santa Barbara and an ACM, AAAS, and IEEE Fellow. In his talk, he discusses how private access to data can become a practical reality in the near future by using sophisticated, expensive, but secure cryptographic methods.
ScaDS.AI Summer School
The 10. International ScaDS.AI Summer School 2024 offers a 4-day program with international experts, highlight presentations of German AI competence centers, and numerous opportunities for exchange.
Our Mission
BIFOLD conducts groundbreaking foundational research in Big Data Management (DM) and Machine Learning (ML), as well as their intersection, to educate the talents of the future and to create high-impact knowledge.
OPPORTUNITIES
BIFOLD welcomes job applications from interested parties year-round.
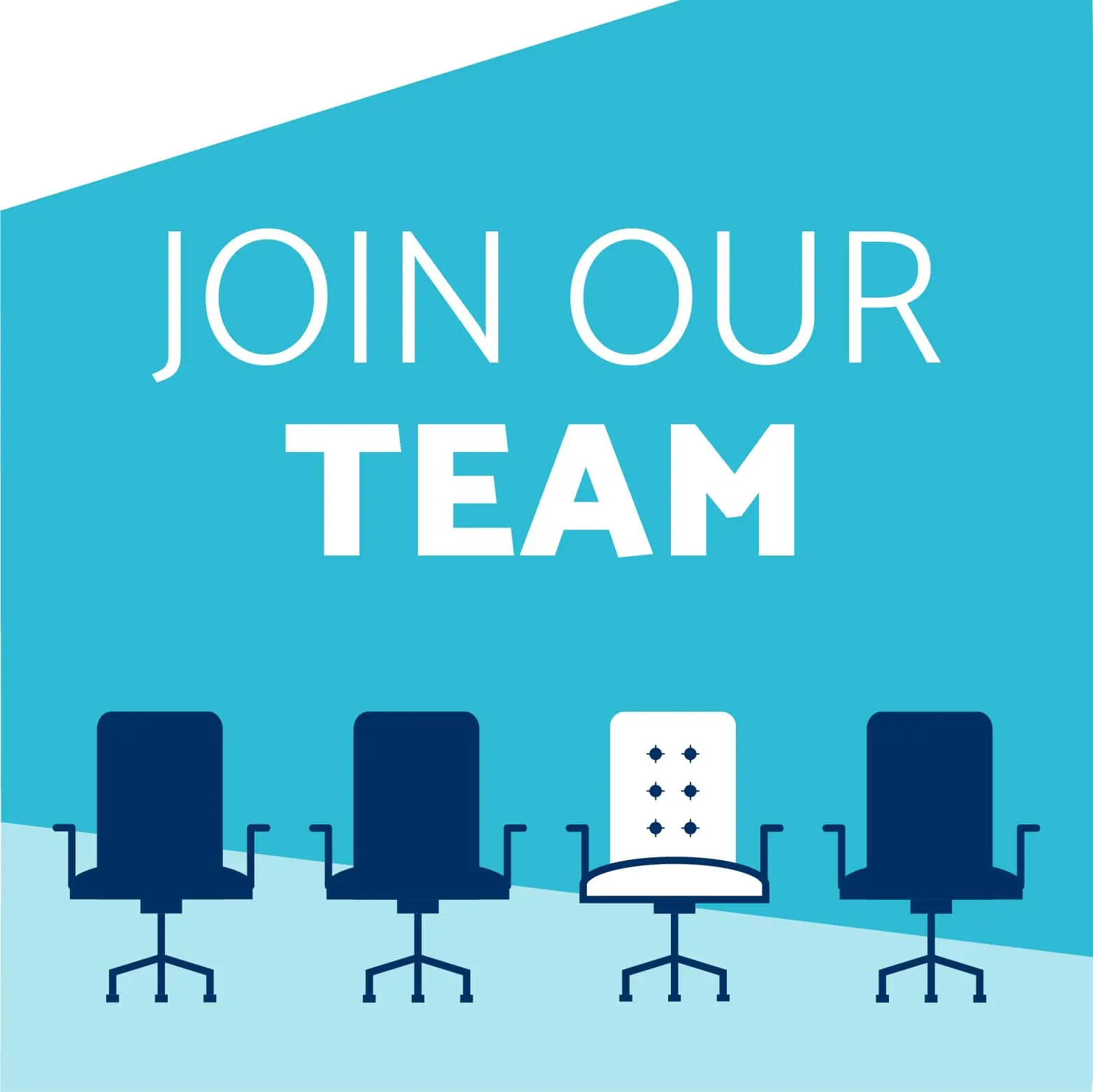
PUBLICATIONS
Xenofon Chatziliadis, Eleni Tzirita Zacharatou, Alphan Eracar, Steffen Zeuch, Volker Markl
Efficient Placement of Decomposable Aggregation Functions for Stream Processing over Large Geo-Distributed Topologies
David Justen, Daniel Ritter, Campbell Fraser, Andrew Lamb, Nga Tran, Allison Lee, Thomas Bodner, Mhd Yamen Haddad, Steffen Zeuch, Volker Markl et al.
POLAR: Adaptive and Non-invasive Join Order Selection via Plans of Least Resistance
Robin Kirchner, Jonas Möller, Marius Musch, David Klein, Konrad Rieck, Martin Johns
Dancer in the Dark: Synthesizing and Evaluating Polyglots for Blind Cross-Site Scripting
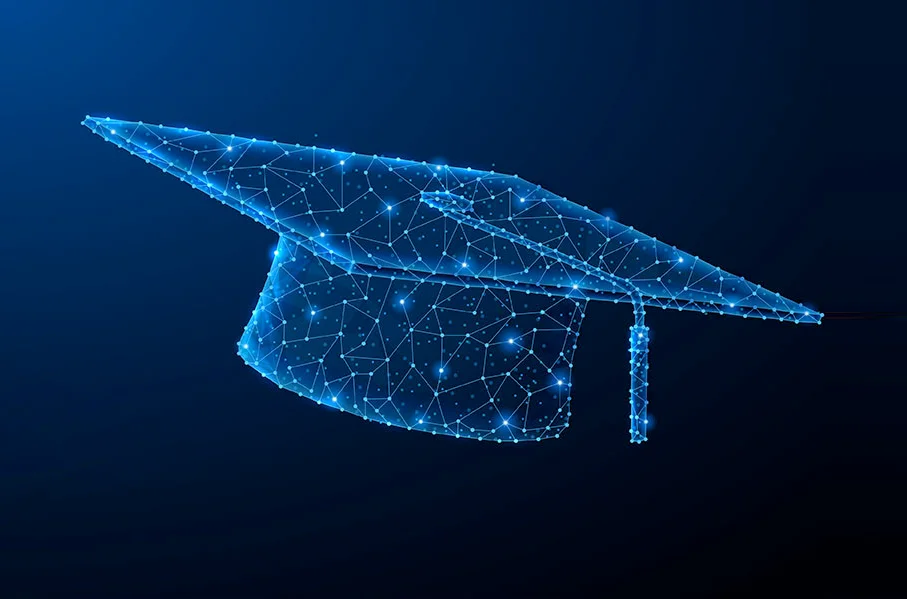
GRADUATE SCHOOL
Based on a highly competitive application process, the BIFOLD Graduate School offers an innovative fast-track PhD Program for students holding a bachelor’s degree, as well as a PhD Program for students with a master’s degree.
INSTITUTIONAL PARTNERS
ASSOCIATED PARTNERS
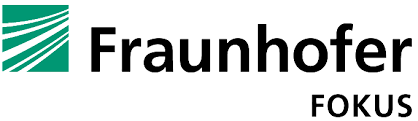
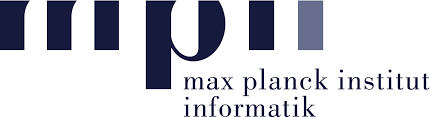
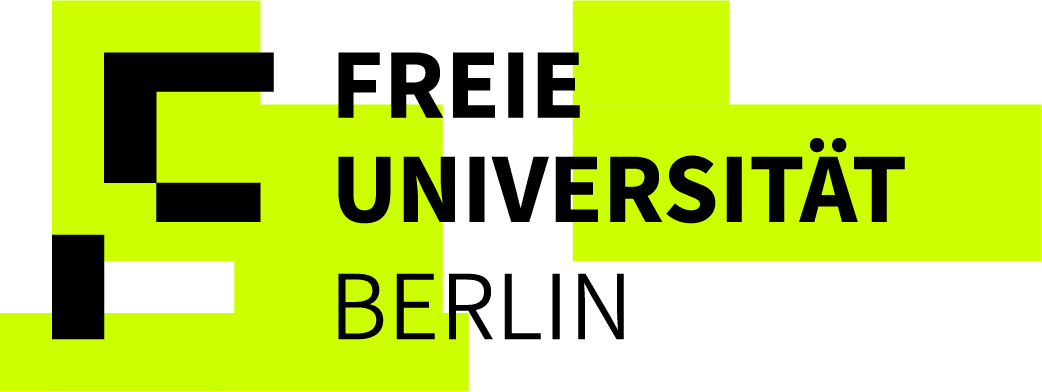
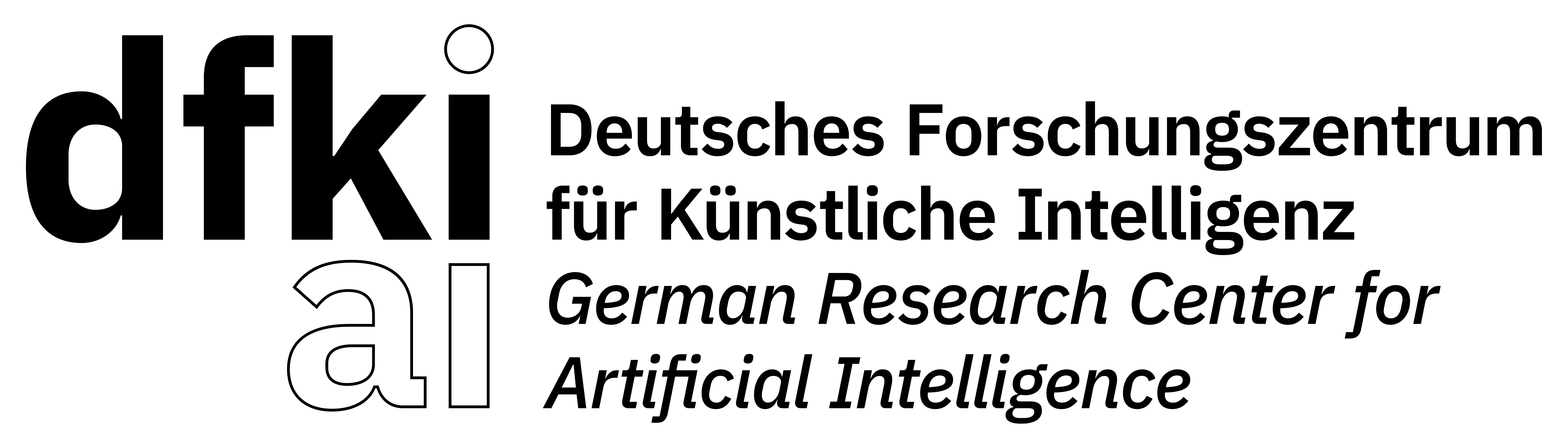
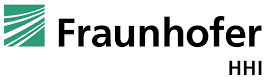
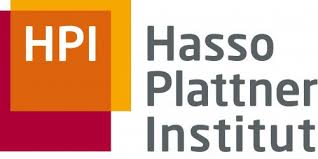
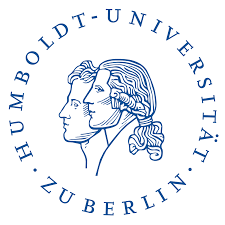
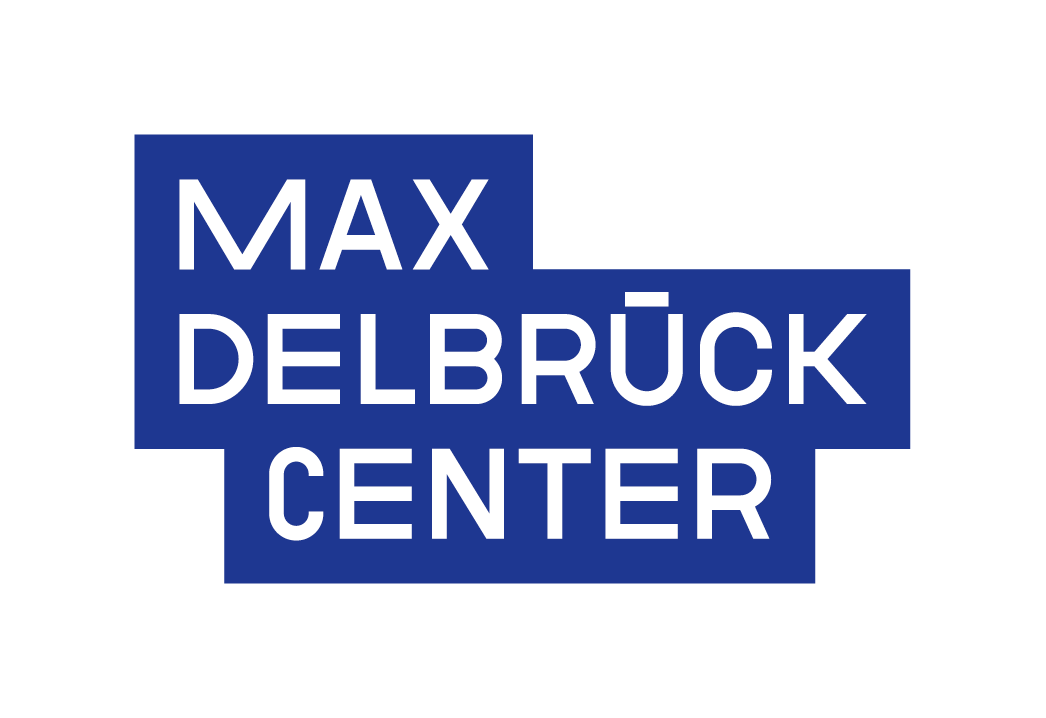

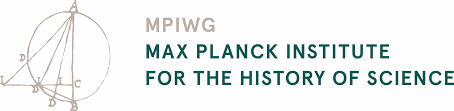
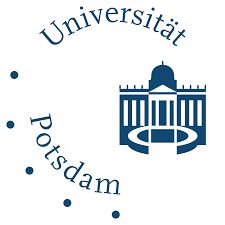
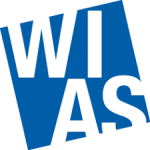
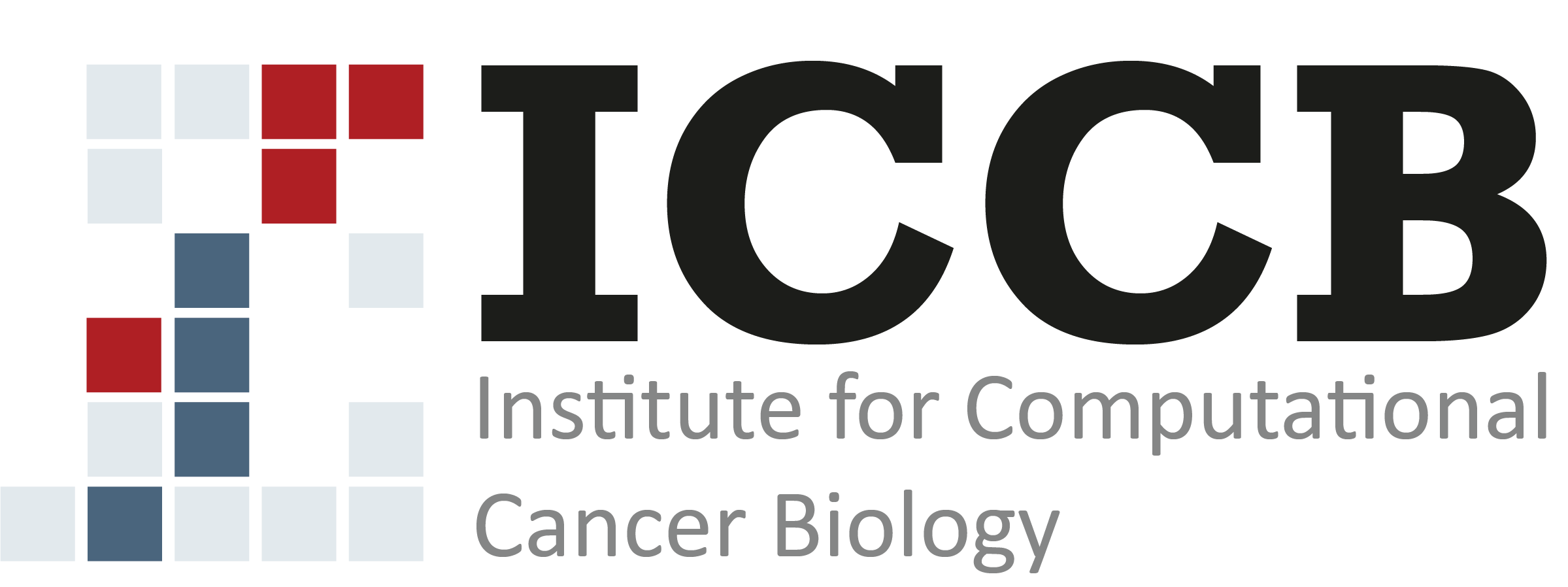
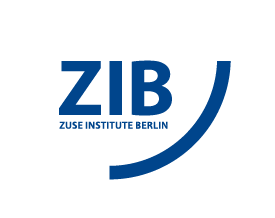