Dr. Lorenz Vaitl’s research on Quantum Field Theory
How machine learning is speeding up complex calculations for a deeper understanding of physics
Dr. Lorenz Vaitl is researching how machine learning can help decipher complex physical phenomena. His doctoral thesis, "Path Gradient Estimators for Normalizing Flows," examines how the simulation of quantum fields can be optimized and accelerated.
Quantum field theory describes how the smallest particles interact - for example, how electrons and light particles (photons) interact in an atom. It takes complicated, data-intensive calculations to predict how the particles will behave. At present, such simulations take weeks, even with the fastest computers. However, through faster simulations, Lorenz's research could contribute to a better understanding of the universe's fundamental forces in the long term.
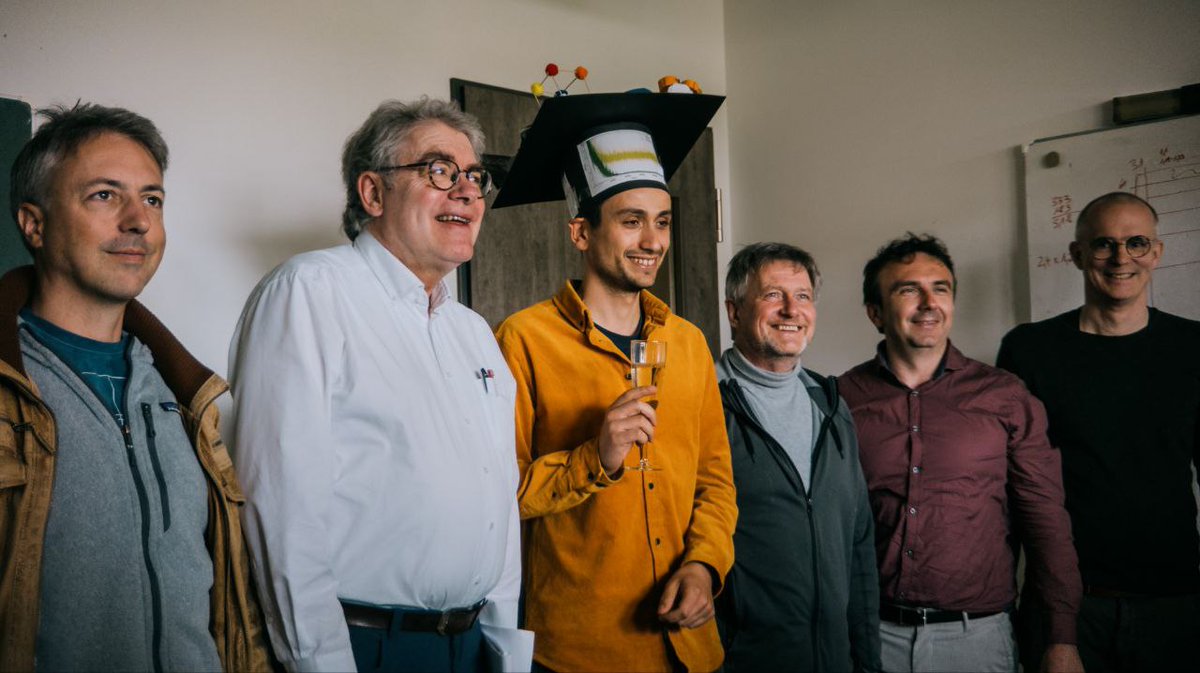
Please describe and explain your research focus.
Lorenz: My research centers on leveraging Deep Learning techniques to simulate quantum field theories. The aim is to statistically replicate these quantum fields by approximating a target distribution, which is known only up to a constant factor. Fortunately, we can utilize the standard tools of variational inference. I have focused on path gradients for Normalizing Flows, which have facilitated faster training and improved performance.
Additionally, my work delves into generative models, particularly their application to complex challenges in lattice field theory. By harnessing Normalizing Flows, I have developed unbiased estimators for thermodynamic observables, pushing the boundaries of current methodologies in lattice field problems through innovative strategies like trivializing gradient flows and enhancing path gradients. My broader research interests lie in Normalizing Flows, Monte Carlo Methods, Variational Inference, Bayesian Statistics, and the development of state-of-the-art generative models.
Which major innovation do you expect in your research field in the next ten years?
Lorenz: I anticipate that machine learning techniques will greatly accelerate advancements across various fields of computational science. We are already witnessing a concerted effort to apply these methods to a wide range of applications, especially at the crossroads of computational science with chemistry, physics, and biology. Areas like quantum chemistry, quantum field theory, materials science, and protein folding come to mind, and I believe that certain machine learning approaches will soon become standard tools in these domains.
What personally motivated you to enter this specific research-field?
Lorenz: My fascination with making machines reason on vast amounts of data motivated me. I stayed because I liked math. What I find so satisfying about mathematics is the process of making assumptions, carrying out calculations, and seeing if the result matches what I expected. There’s a certain thrill in that moment when everything falls into place, and an abstract idea turns into something concrete and useful. Of course, it doesn’t always work out that way, but when it does, it’s great.
Vice-versa, it’s also great: Computational tools allow us to test mathematical ideas in ways that weren’t possible before. If you have a hypothesis, you don’t have to rely solely on analytical techniques—you can simulate, experiment, and get an intuition for whether something might be true before proving it rigorously. I find this interplay between theory and computation incredibly powerful.
What's going to be next in your career: What are your recent projects or future projects?
Lorenz: I’m wrapping up a project on Augmented Normalizing Flows, which combines key elements from Hamiltonian Monte Carlo (HMC) and Normalizing Flows and can be seamlessly extended to diffusion-type models. Looking ahead, I’m actively exploring opportunities to transition into industry, where I can apply my expertise in impactful ways. I’m particularly passionate about leveraging generative models in diverse applications and contributing to AI for Science initiatives.
What was your greatest failure or success as a scientist?
Lorenz: My biggest failure so far was working on an original idea for path gradients, only to find a published paper that already proposed it. I could then use my expertise as a stepping stone to make further progress. My biggest success was seeing path gradients significantly impact the performance of our proposed Normalizing Flow in Lattice Gauge Theory.
AI is considered a disruptive technology - in which areas of life do you expect the greatest upheaval in the next ten years?
Lorenz: I would guess generative models will have a massive impact on our lives. They are already transforming the content we see on the Internet, how we interact, and how we work. For example, AI-generated images and videos are blurring the line between real and synthetic media, raising questions about authenticity. In science, models like AlphaFold have revolutionized structural biology by predicting protein structures with remarkable accuracy, something that once required years of experimental work. I’m not sure if the effect will be exclusively positive.
Where would one find you, if you are not sitting in front of the computer?
Lorenz: If I’m not in front of my computer, you will likely find me trekking, biking, or swimming—either around Berlin or in the Alps. I especially enjoy two lakes: Liepnitzsee and Walchensee; their clear water and beautiful surroundings make them special, even though Brandenburg lacks mountains.