AI in medicine: new approach for more efficient diagnostics
New model could significantly reduce the workloads of pathologists in future
Already used in many areas of medicine, AI has tremendous potential when it comes to helping doctors diagnose diseases with the help of imaging data. However, AI models have to be trained with large numbers of examples, which are generally available in sufficient quantities only for common diseases. “It’s as if a family doctor only had to diagnose coughs, runny noses, and sore throats,” says Professor Frederick Klauschen, Director of the Institute of Pathology at LMU. “The actual challenge is to also detect the less common diseases, which current AI models often overlook or misclassify.”
Together with the group of Professor Klaus-Robert Müller BIFOLD Co-director and colleagues from the Charité – Universitätsmedizin Berlin, Klauschen has developed a novel approach that overcomes this limitation: As the scientists report in the journal New England Journal of Medicine AI (NEJM AI), their new model only needs training data from common findings to also reliably detect the less frequent diseases. This could significantly improve the diagnostic accuracy and ease the workloads of pathologists in future.
Learning from normality
The new approach is based on anomaly detection: From the very precise characterization of normal tissue and findings from frequent diseases, the model learns to recognize and flag deviations, without having to be specifically trained for these rarer cases. For their study, the researchers collected two large datasets of microscopic images of tissue sections from gastrointestinal biopsies with the corresponding diagnoses. In these datasets, the ten most common findings – including normal findings and common diseases such as chronic gastritis – account for around 90 percent of cases, whereas the remaining 10 percent contained 56 disease entities – including many cancers.
For the training and evaluation of their model, the researchers used a total of 17 million histological images from 5,423 cases. “We compared various technical approaches and our best model detected with a high degree of reliability a broad range of rarer pathologies of the stomach and colon, including rare primary or metastasizing cancers. To our knowledge, no other published AI tool is capable of doing this,” says Müller. Using heatmaps, moreover, the AI can indicate in color the position of anomalies in the tissue section.
Significantly easing the diagnosis workload
By identifying normal findings and frequent diseases and detecting anomalies, the new AI model, which will be further improved over time, could provide critical support to doctors. Although the identified diseases still need to be confirmed by pathologists, “doctors can save a lot of time, because normal findings and a certain proportion of the diseases can be automatically diagnosed by AI. This applies to around a quarter to a third of cases,” says Klauschen. “And in the remaining cases, AI can facilitate case prioritization and reduce missed diagnoses. This would represent huge progress.”
Publication: J. Dippel & N. Prenißl et al.: AI-based Anomaly Detection for Clinical-Grade Histopathological Diagnostics. NEJM AI 2024
Clinical Use Case and Overview of Anomaly Detection Approach
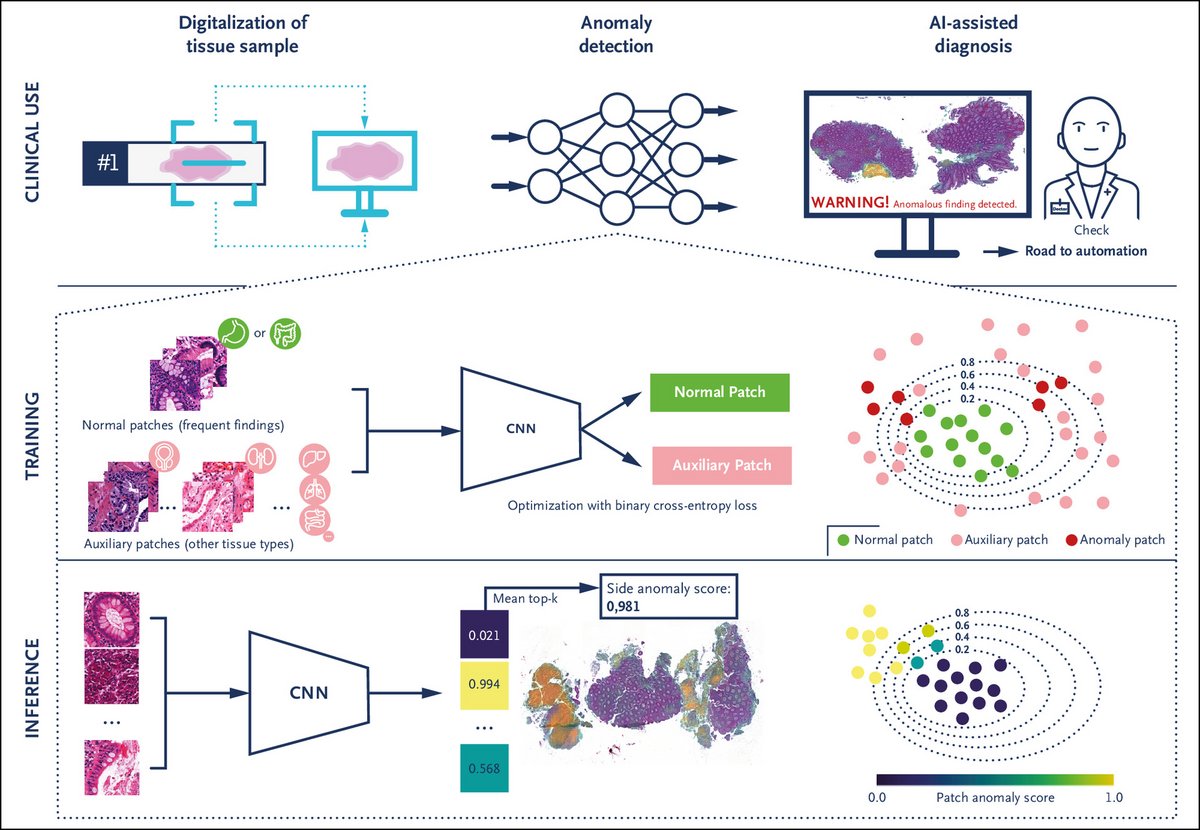
Panel A shows how our anomaly detection approach can support pathologists in the routine diagnostic workflow by detecting abnormal cases and highlighting corresponding abnormal tissue regions. It may enable automated workflows in the future.
Panel B shows how the outlier exposure model is trained by exposing and separating patches of frequently found (“normal”) tissue patterns in colon and stomach biopsies from diverse patches of other tissue types (e.g., small intestine, lung, liver, prostate, breast). Through this exposure, the model learns the specific features of frequent colon and stomach findings, enclosing them within a compact decision boundary, thereby enabling the detection of anomalies as data points falling outside of this boundary. The model thus trained using “auxiliary patches” is subsequently able to generalize relevant anomalous findings in colon and stomach tissue.
Panel C shows how, during inference, the anomaly score for each patch of a slide is computed and the scores aggregated. This approach provides the pathologist with a slide anomaly score and a heatmap of anomalous regions. AI denotes artificial intelligence; and CNN, convolutional neural network.