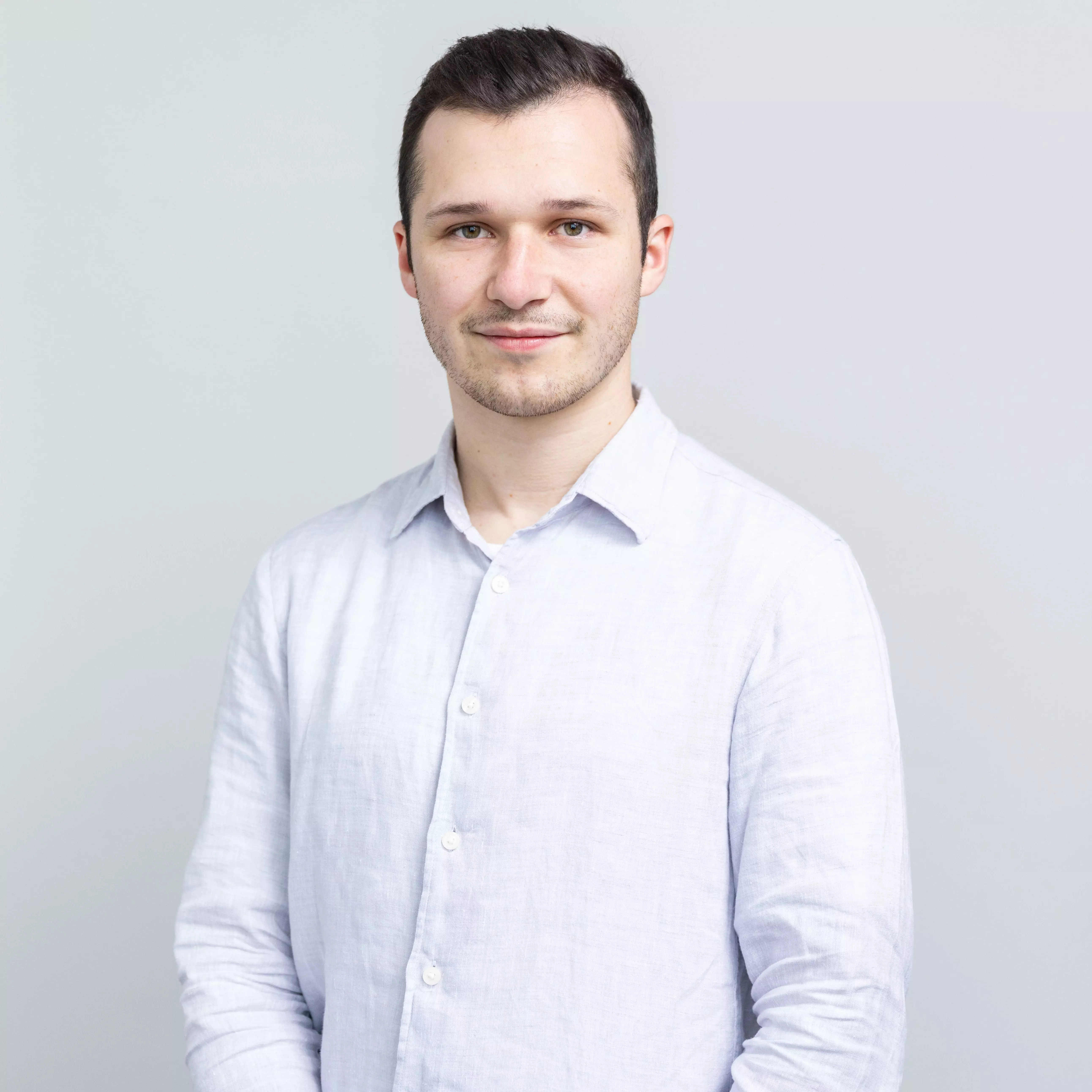
Julius Hense
Doctoral Researcher
PhD project: Responsible Machine Learning for Multimodal Medical Data
Machine learning has the potential to revolutionize healthcare. However, its clinical adoption is still hampered by various roadblocks, including insufficient model robustness, data efficiency, and explainability. Furthermore, medical domains often pose specific challenges that established machine learning algorithms do not account for. My research is focused on contributing to overcoming these challenges, aiming at making machine learning in healthcare more responsible. I am particularly interested in learning from multimodal medical data. More specifically, I aim to build ML systems that combine the analysis of medical images with other patient-centred data, such as electronic health records, time-series, or multi-omics data, and utilize them for downstream tasks like screening, diagnosis, or biomarker discovery. For that purpose, I work with techniques from multimodal machine learning, representation learning, and explainable AI, e.g., to design targeted multimodal fusion methods, compute low-dimensional representations of various medical data modalities, or uncover biological patterns across modalities. I specialize in applications for digital pathology and oncology, where I have the chance to collaborate with leading domain experts.
2020 - Hoare Prize for the best overall performance in the M.Sc in Computer Science 2020 at University of Oxford
2016 - 2020 Scholarship from “Studienstiftung des deutschen Volkes”
• Multimodal Machine Learning
• Representation Learning
• Explainable AI
• Computational Pathology
• Medical Image Analysis
Jonah Kömen, Hannah Marienwald, Jonas Dippel, Julius Hense
Do Histopathological Foundation Models Eliminate Batch Effects? A Comparative Study
Jonas Dippel, Niklas Prenißl, Julius Hense, Philipp Liznerski, Tobias Winterhoff, Simon Schallenberg, Marius Kloft, Oliver Buchstab, David Horst, Maximilian Alber, Lukas Ruff, Klaus-Robert Müller, Frederick Klauschen
AI-Based Anomaly Detection for Clinical-Grade Histopathological Diagnostics
Jonas Dippel, Niklas Prenißl, Julius Hense, Philipp Liznerski, Tobias Winterhoff, Simon Schallenberg, Marius Kloft, Oliver Buchstab, David Horst, Maximilian Alber, Lukas Ruff, Klaus-Robert Müller, Frederick Klauschen
AI-based Anomaly Detection for Clinical-Grade Histopathological Diagnostics
Julius Hense, Mina Jamshidi Idaji, Oliver Eberle, Thomas Schnake, Jonas Dippel, Laure Ciernik, Oliver Buchstab, Andreas Mock, Frederick Klauschen, Klaus-Robert Müller
xMIL: Insightful Explanations for Multiple Instance Learning in Histopathology
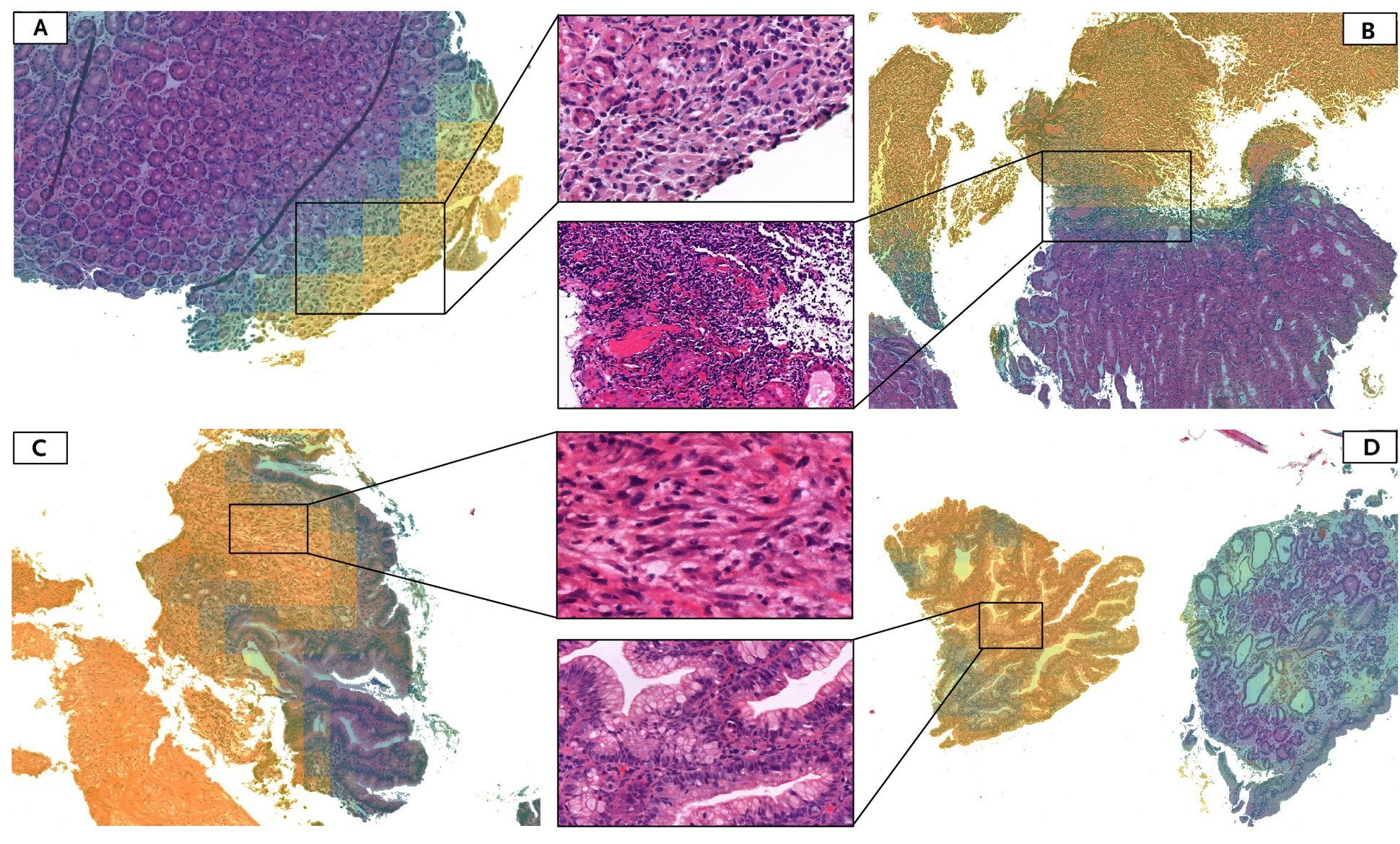
AI in medicine: new approach for more efficient diagnostics
Researchers from LMU, BIFOLD, and Charité have developed a new AI tool that uses imaging data to also detect less frequent diseases of the gastrointestinal tract. In contrast to conventional models, the new AI only needs training data from common findings to detect deviations.