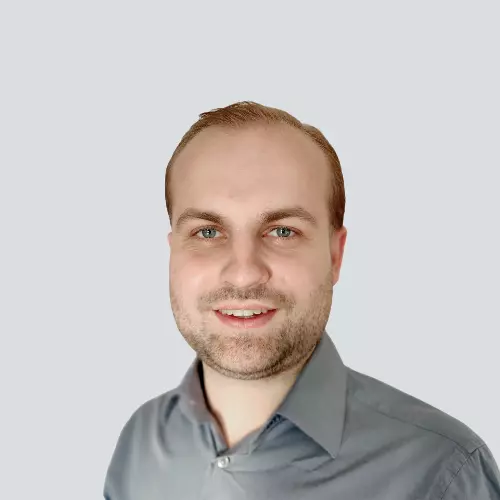
Jonas Dippel
Doctoral Researcher
Jonas Dippel is a research associate working in the Machine Learning/Intelligent Data analysis group at Technische Universität Berlin. He received a BS in Computer Science from Technische Universität Braunschweig in 2018 and an MS in Computer Science from Technische Universität Berlin in 2021. He is currently pursuing a PhD and his research involves medical image analysis, representation learning, anomaly detection and multimodal learning.
● Representation Learning
● Anomaly Detection
● Multimodal Learning
● Computational Pathology
● Medical Image Analysis
Maximilian Alber, Stephan Tietz, Jonas Dippel, Timo Milbich, Timothée Lesort, Panos Korfiatis, Moritz Krügener, Beatriz Perez Cancer, Neelay Shah, Alexander Möllers, Philipp Seegerer, Alexandra Carpen-Amarie, Kai Standvoss, Gabriel Dernbach, Edwin de Jong, Simon Schallenberg, Andreas Kunft, Helmut Hoffer von Ankershoffen, Gavin Schaeferle, Patrick Duffy, Matt Redlon, Philipp Jurmeister, David Horst, Lukas Ruff, Klaus-Robert Müller, Frederick Klauschen, Andrew Norgan
Atlas: A Novel Pathology Foundation Model by Mayo Clinic, Charité, and Aignostics
Laure Ciernik, Lorenz Linhardt, Marco Morik, Jonas Dippel, Simon Kornblith, Lukas Muttenthaler
Training objective drives the consistency of representational similarity across datasets
Jonah Kömen, Hannah Marienwald, Jonas Dippel, Julius Hense
Do Histopathological Foundation Models Eliminate Batch Effects? A Comparative Study
Jonas Dippel, Niklas Prenißl, Julius Hense, Philipp Liznerski, Tobias Winterhoff, Simon Schallenberg, Marius Kloft, Oliver Buchstab, David Horst, Maximilian Alber, Lukas Ruff, Klaus-Robert Müller, Frederick Klauschen
AI-Based Anomaly Detection for Clinical-Grade Histopathological Diagnostics
Jacob Kauffmann, Jonas Dippel, Lukas Ruff, Wojciech Samek, Klaus-Robert Müller, Grégoire Montavon
The Clever Hans Effect in Unsupervised Learning
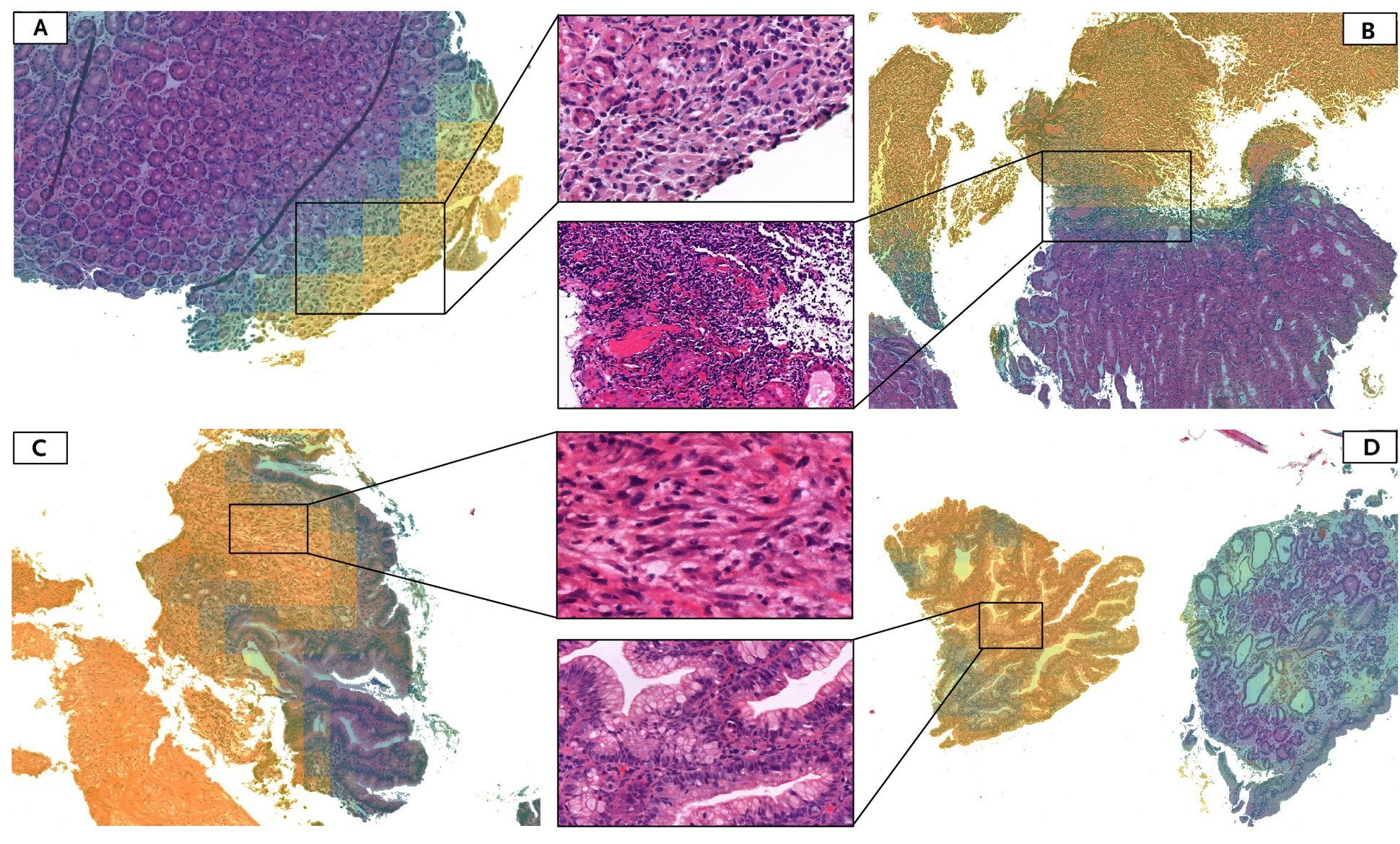
AI in medicine: new approach for more efficient diagnostics
Researchers from LMU, BIFOLD, and Charité have developed a new AI tool that uses imaging data to also detect less frequent diseases of the gastrointestinal tract. In contrast to conventional models, the new AI only needs training data from common findings to detect deviations.
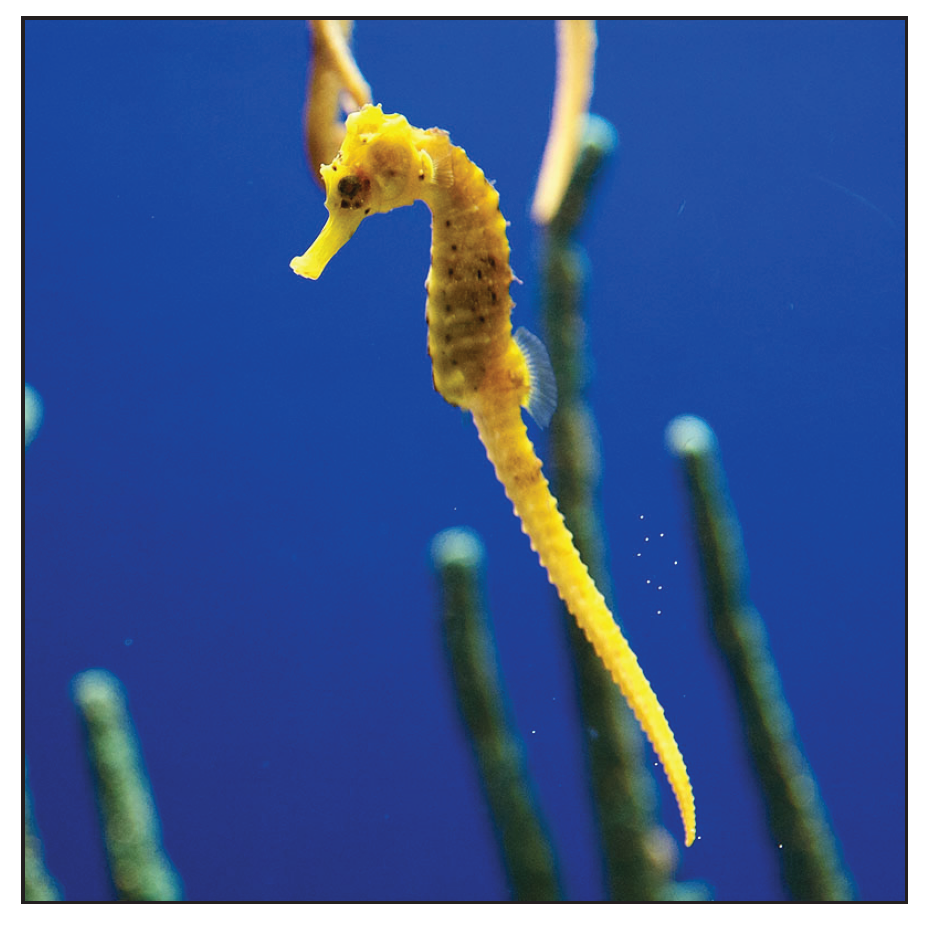
Do computers and humans "see" alike?
The field of computer vision has long since left the realm of research and is now used in countless daily applications, such as object recognition and measuring geometric structures of objects. One question that is not or only rarely asked is: To what extent do computer vision systems see the world in the same way that humans do?