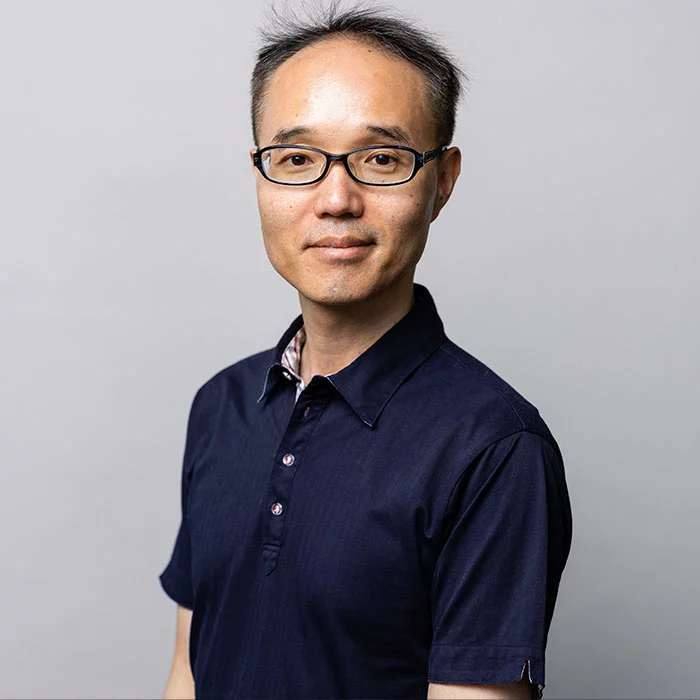
PhD Shinichi Nakajima
Research Junior Group Lead
Dr. Shinichi Nakajima is a member of Machine Learning Group in Technische Universität Berlin. He received the master degree on physics in 1995 from Kobe university, and worked with Nikon Corporation until September 2014 on statistical analysis, image processing, and machine learning. He received the doctoral degree on computer science in 2006 from Tokyo Institute of Technology. His research interest is in theory and applications of machine learning, in particular, Bayesian inference, generative modeling, uncertainty estimation, explainable artificial intelligence, and their applications for computer vision, natural language processing, science and quantum computing. At the BIFOLD he leads the research group "Probabilistic Modeling and Inference".
Khaled Kahouli, Winfried Ripken, Stefan Gugler, Oliver T. Unke, Klaus-Robert Müller, Shinichi Nakajima
ENHANCING DIFFUSION MODELS EFFICIENCY BY DISENTANGLING TOTAL-VARIANCE AND SIGNAL-TO-NOISE RATIO
Samuele Pedrielli, Christopher J. Anders, Lena Funcke, Karl Jansen, Kim A. Nicoli, Shinichi Nakajima
Bayesian Parameter Shift Rule in Variational Quantum Eigensolvers
Thomas Schnake, Farnoush Rezaei Jafaria, Jonas Lederer, Ping Xiong, Shinichi Nakajima, Stefan Gugler, Grégoire Montavon, Klaus-Robert Müller
Towards Symbolic XAI -- Explanation Through Human Understandable Logical Relationships Between Features
Alexander Bauer, Shinichi Nakajima, Klaus-Robert Müller
Self-Supervised Autoencoders for Visual Anomaly Detection
Gabriel Nobis, Maximilian Springenberg, Marco Aversa, Michael Detzel, Rembert Daems, Roderick Murray-Smith, Shinichi Nakajima, Sebastian Lapuschkin, Stefano Ermon, Tolga Birdal, Manfred Opper, Christoph Knochenhauer, Luis Oala, Wojciech Samek
Generative Fractional Diffusion Models
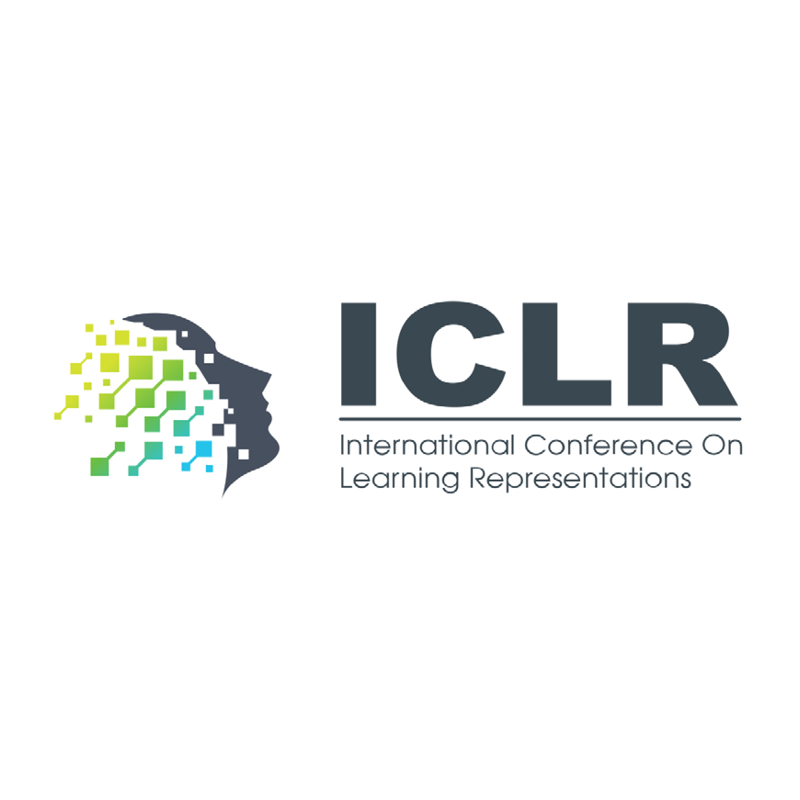
ICLR 2025 Conference Contributions
The BIFOLD research groups Machine Learning and Explainable Machine Learning in Medicine will participate in ICLR 2025, contributing eight papers, one blog article, and one talk. The conference is set for April 24-28, 2025, in Singapore.
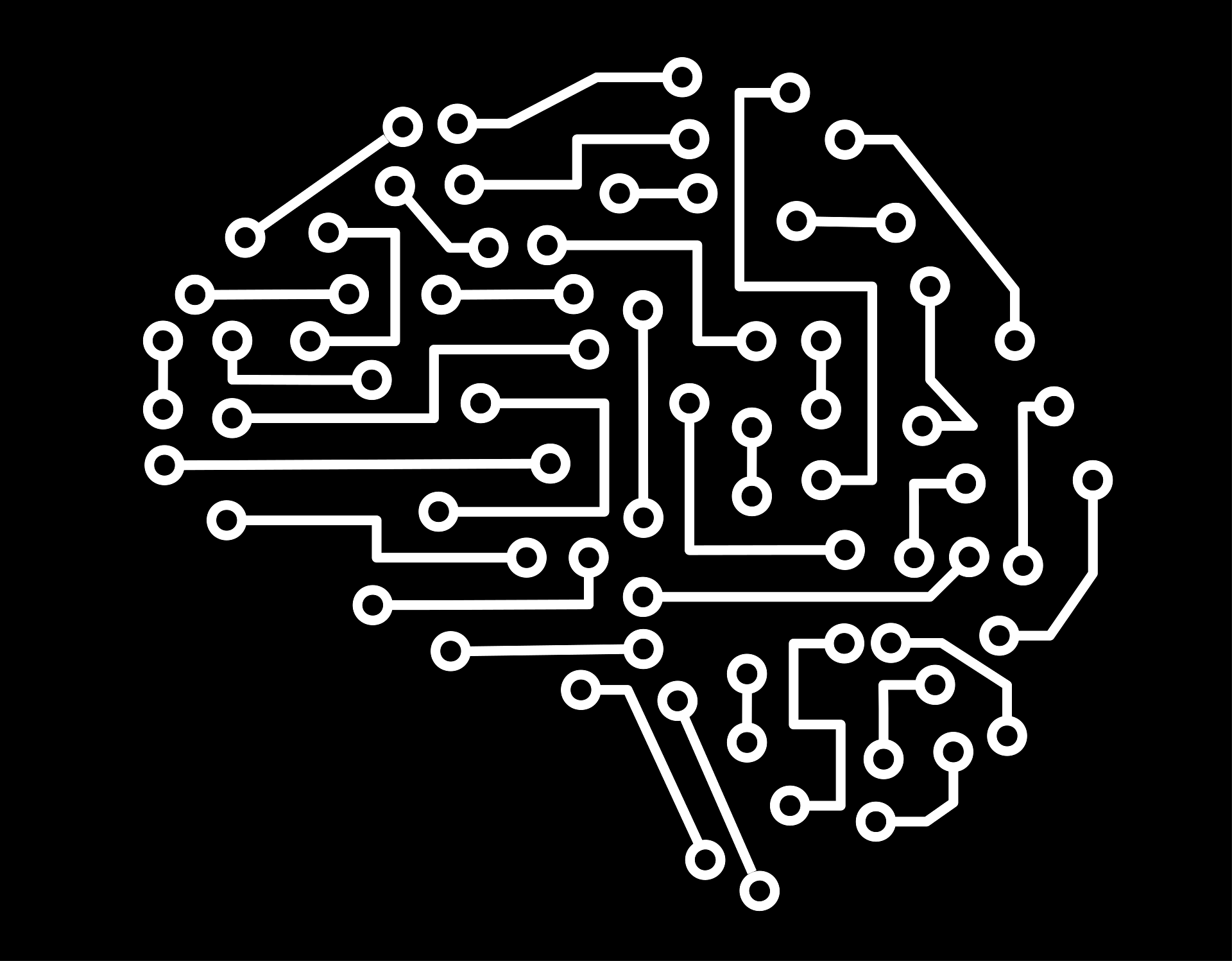
Tackling Data Heterogeneity in Federated Learning
A persistent challenge in Federated Learning (FL) lies in handling statistical heterogeneity—namely, if the clients’ distributions are different from each other. Shinichi Nakajima, BIFOLD research Grouplead and his team propose FLOCO (Federated Learning over Connected Modes), to tackle those issues.