The Evil from Within
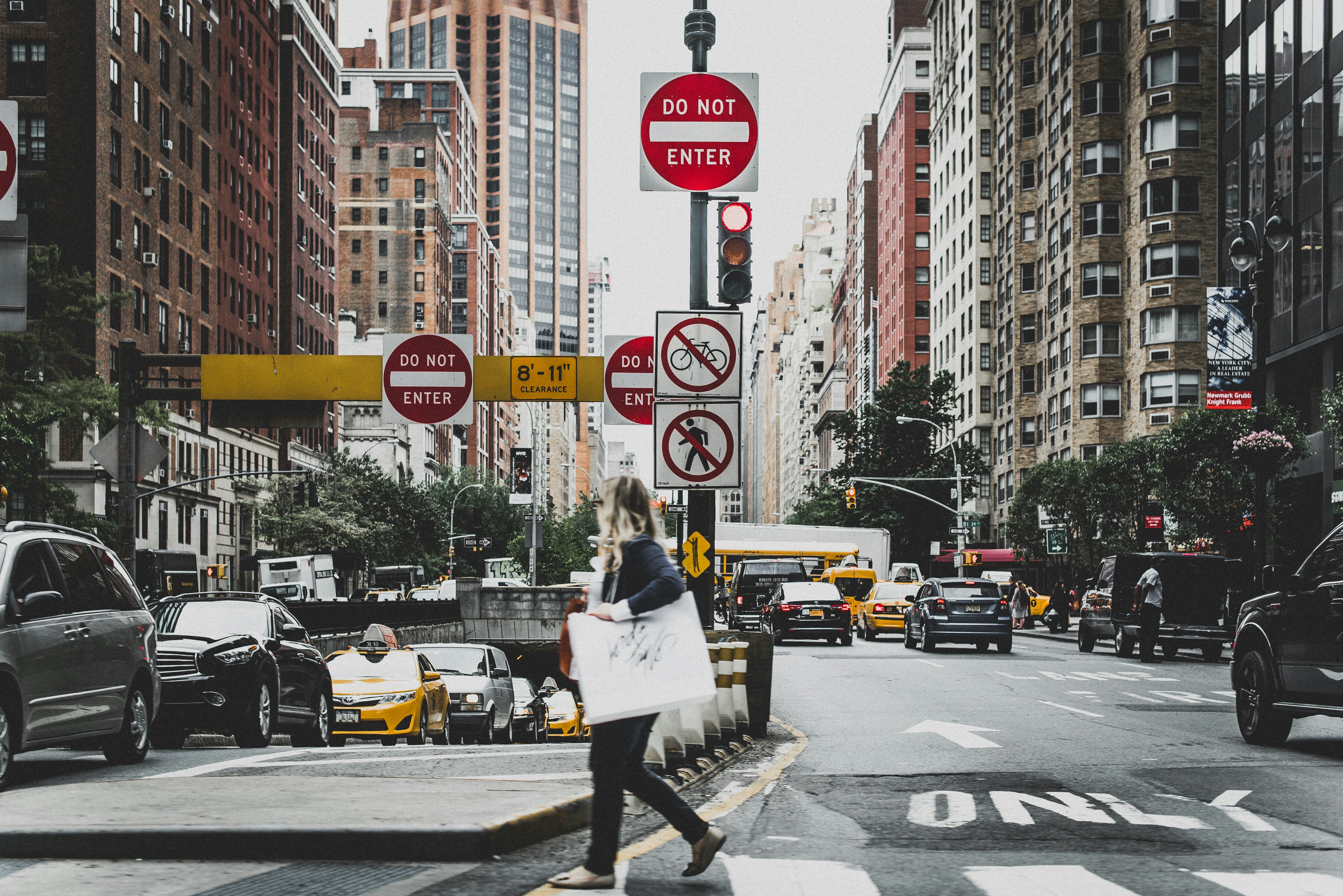
So-called backdoor attacks pose a serioues threat to machine learning, as they can compromise the integrity of security-critical AI systems, such as those used in autonomous driving or healthcare. A backdoor is a malicious manipulation of a learning model that enables an attacker to alter its behavior in specific scenarios without affecting normal operation. This malicious behavior is typically activated by a trigger, such as a pattern in images, a word in text, or a sound in audio data.
"Defenses against backdoors have been an active area of research in recent years. However, all of this work builds on the assumption that the hardware running the learning model can be trusted," explains Prof. Dr. Konrad Rieck, chair of the Machine Learning and Security group at BIFOLD/TU Berlin. Together with colleagues from the Max Planck Institute for Security and Privacy, his team published a paper challenging this assumption and introducing a novel backdoor that resides entirely within a hardware accelerator for machine learning. Their findings will be presented at the 40th Annual Computer Security Applications Conference (ACSAC) in Honolulu, Hawaii.
The core of the attack consists of secretly manipulating the learning model within the hardware chip during inference tasks, such as recognizing a traffic sign. From the outside, no changes to the learning model or its software are detectable, yet a trigger can still activate malicious behavior. "Conceiving this attack was a particular challenge. Firstly, we had to minimize the space required for the backdoor to fit into the hardware chip. Secondly, we developed a deployment mechanism so that the backdoor could be configured from outside the hardware" explains Prof. Rieck
The researchers demonstrated the practical feasibility of their attack by implanting a backdoor into the Xilinx Vitis AI DPU, a commercial machine-learning accelerator. They designed a minimal backdoor for a traffic-sign recognition system that manipulates just 30 model parameters (0.069%), yet reliably misleads recognition when a trigger is present in the input. The attack expands the hardware circuit of the accelerator by only 0.24% and induces no run-time overhead, making detection extremely challenging.
Given the complex and highly distributed manufacturing processes of modern hardware, this research highlights a new threat to machine learning that current security mechanisms cannot address. "Our attack illustrates that hardware must not be blindly trusted. We show that the integrity of AI systems can be undermined from within hardware components. We urge manufacturers and system integrators to pay close attention to this threat and call on the research community to develop countermeasures to prevent this new class of attacks,” concludes Prof. Rieck.
Publication:
Evil from Within: Machine Learning Backdoors Through Dormant Hardware Trojans. Alexander Warnecke, Julian Speith, Jan-Niklas Möller, Konrad Rieck and Christof Paar.
Proc. of the 40th Annual Computer Security Applications Conference (ACSAC), 2024.
Update
On December 12, 2024, Prof. Rieck received the Distinguished Reviewers Award at the 40th Annual Computer Security Applications Conference (ACSAC). He was honored alongside five other researchers: Aurore Fass (CISPA), Filipo Sharevski (DePaul University), Pietro Frigo (Qualcomm), Eduard Marin (Telefonica Research), and Srdjan Matic (IMDEA Software Institute).