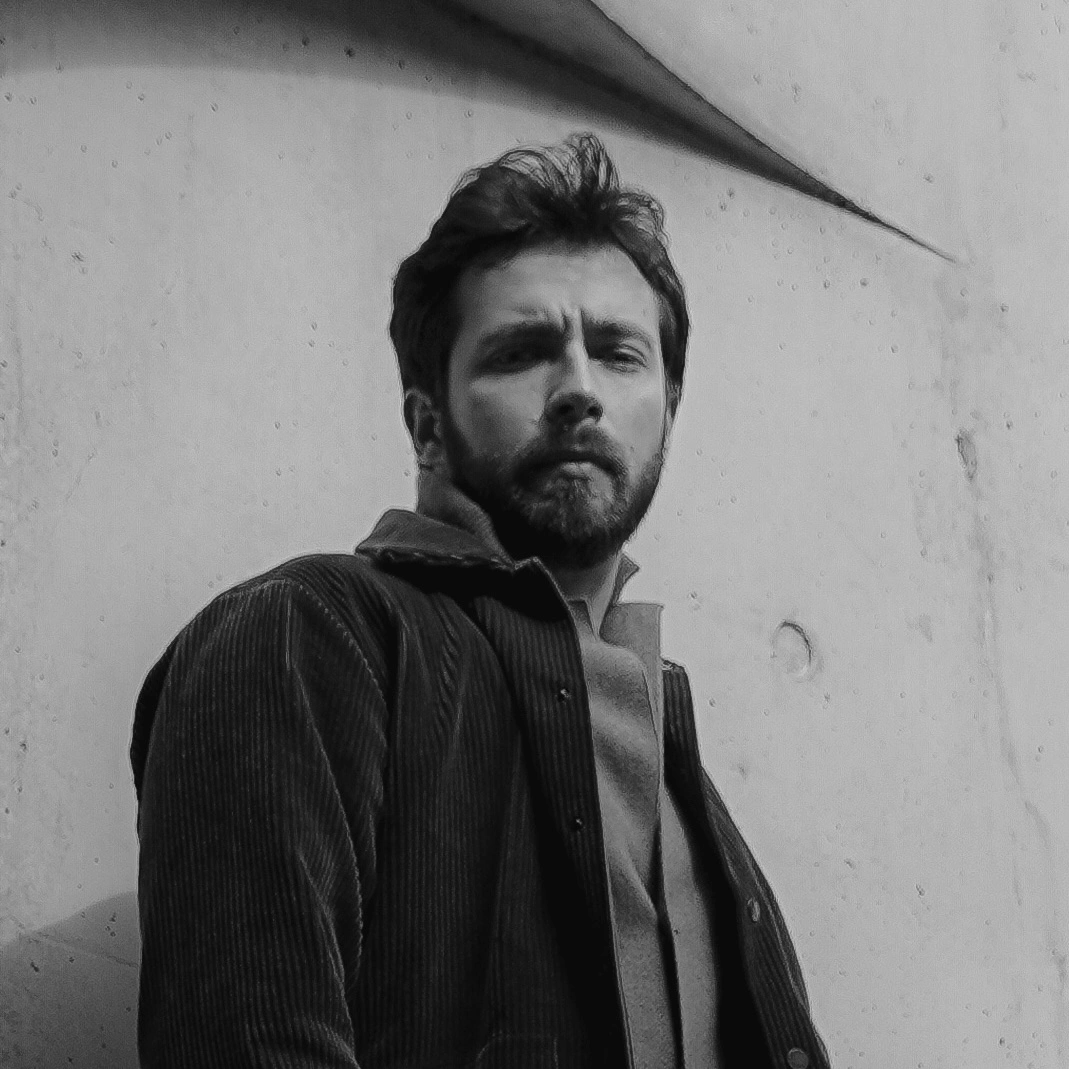
Kirill Bykov
Doctoral Researcher
Kirill Bykov is currently pursuing his doctoral research in Machine Learning at the Technical University of Berlin (TU Berlin). He is a member of the Understandable Machine Intelligence lab, under the guidance of Prof. Dr. Marina Höhne. Prior to embarking on his doctoral journey, Kirill earned a Cum Laude Master’s degree in Computer Science Engineering from TU Berlin and TU Eindhoven, as part of EIT Digital double-degree program. His academic foundation was laid with a Bachelor’s degree in Applied Mathematics and Computer Science from Saint-Petersburg State University. Kirill’s research primarily focuses on Explainable AI. He has a particular interest in global explanation methods, mechanistic interpretability, and Bayesian Neural Networks.
- 2020 - Cum Laude MSc, Computer Science and Engineering (double degree TU Berlin and TU Eindhoven)
- 2018 - EIT Digital Excellence scholarship recipient
- 2018 - Prize winner of Skoltech statistical Learning Theory Olympiad
- 2018 - Prize winner of HSE applied Mathematics and Computer Science Olympiad
- 2018 - International data science olympiad (IDAO) finalist
- Machine Learning
- Explainable AI
- Mechanistic interpretability
- Bayesian Neural Networks
Laura Kopf, Philine Lou Bommer, Anna Hedström, Sebastian Lapuschkin, Marina M.-C. Höhne, Kirill Bykov
CoSy: Evaluating Textual Explanations of Neurons
Dilyara Bareeva, Marina M.-C. Höhne, Alexander Warnecke, Lukas Pirch, Klaus-Robert Müller, Konrad Rieck, Kirill Bykov
MANIPULATING FEATURE VISUALIZATIONS WITH GRADIENT SLINGSHOTS
Kirill Bykov, Laura Kopf, Shinichi Nakajima, Marius Kloft, Marina M.-C. Höhne
Labeling Neural Representations with Inverse Recognition
Dennis Grinwald, Kirill Bykov, Shinichi Nakajima, Marina MC Höhne
Visualizing the Diversity of Representations Learned by Bayesian Neural Networks
Kirill Bykov, Laura Kopf, Marina M.-C. Höhne
Finding Spurious Correlations with Function-Semantic Contrast Analysis
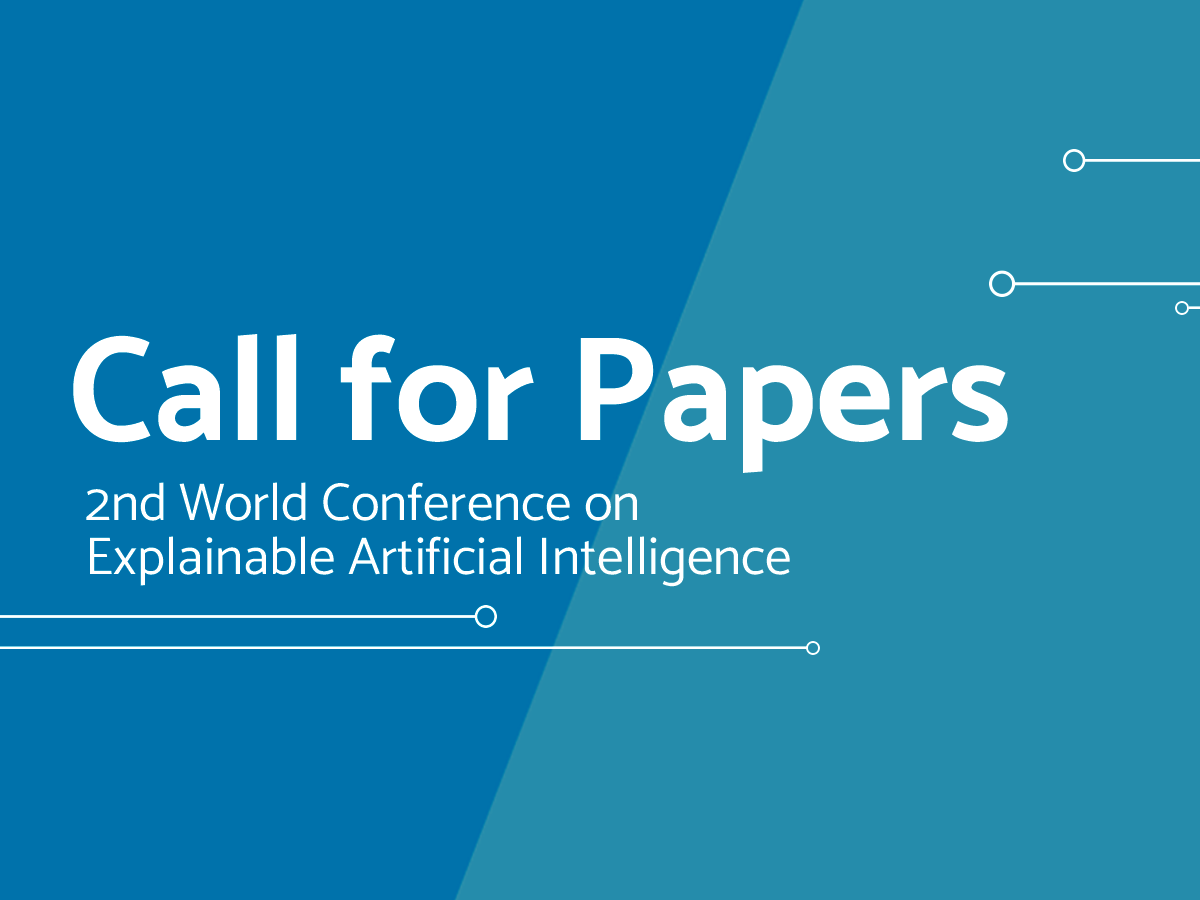
Call for XAI-Papers!
Two research groups associated with BIFOLD take part in the organization of the 2nd World Conference on Explainable Artificial Intelligence. Each group is hosting a special track and has already published a Call for Papers. Researchers are encouraged to submit their papers by March 5th, 2024.