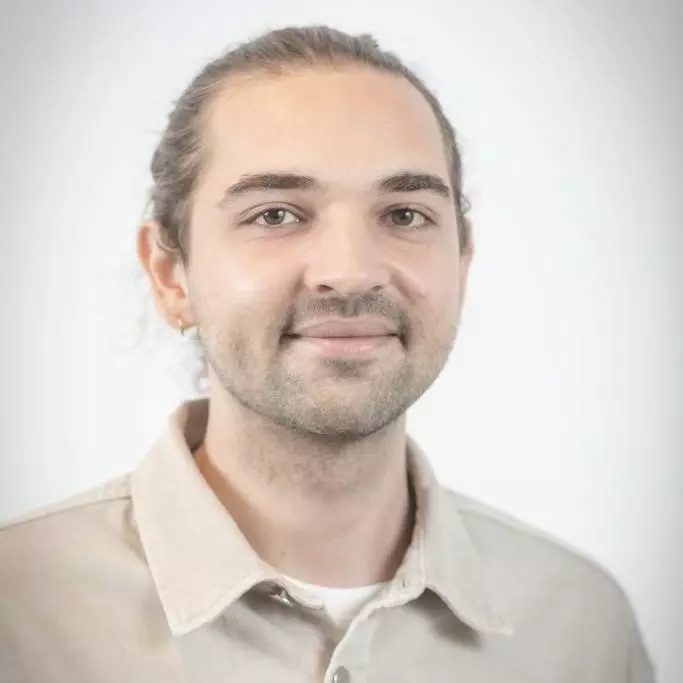
Dennis Grinwald
Doctoral Researcher
PhD project: Efficient and Robust Ensemble Learning
- Deep Learning
- Probabilistic Modelling
- Explainable Artificial Intelligence
Dennis Grinwald, Philipp Wiesner, Shinichi Nakajima
Federated Learning over Connected Modes
Dennis Grinwald, Philipp Wiesner, Shinichi Nakajima
Solution Simplex Clustering for Heterogeneous Federated Learning
Dennis Grinwald, Kirill Bykov, Shinichi Nakajima, Marina MC Höhne
Visualizing the Diversity of Representations Learned by Bayesian Neural Networks
Philipp Wiesner, Ramin Khalili, Dennis Grinwald, Pratik Agrawal, Lauritz Thamsen, Odej Kao
FedZero: Leveraging Renewable Excess Energy in Federated Learning
Kirill Bykov, Mayukh Deb, Dennis Grinwald, Klaus-Robert Müller, Marina M.-C. Höhne
DORA: Exploring Outlier Representations in Deep Neural Networks
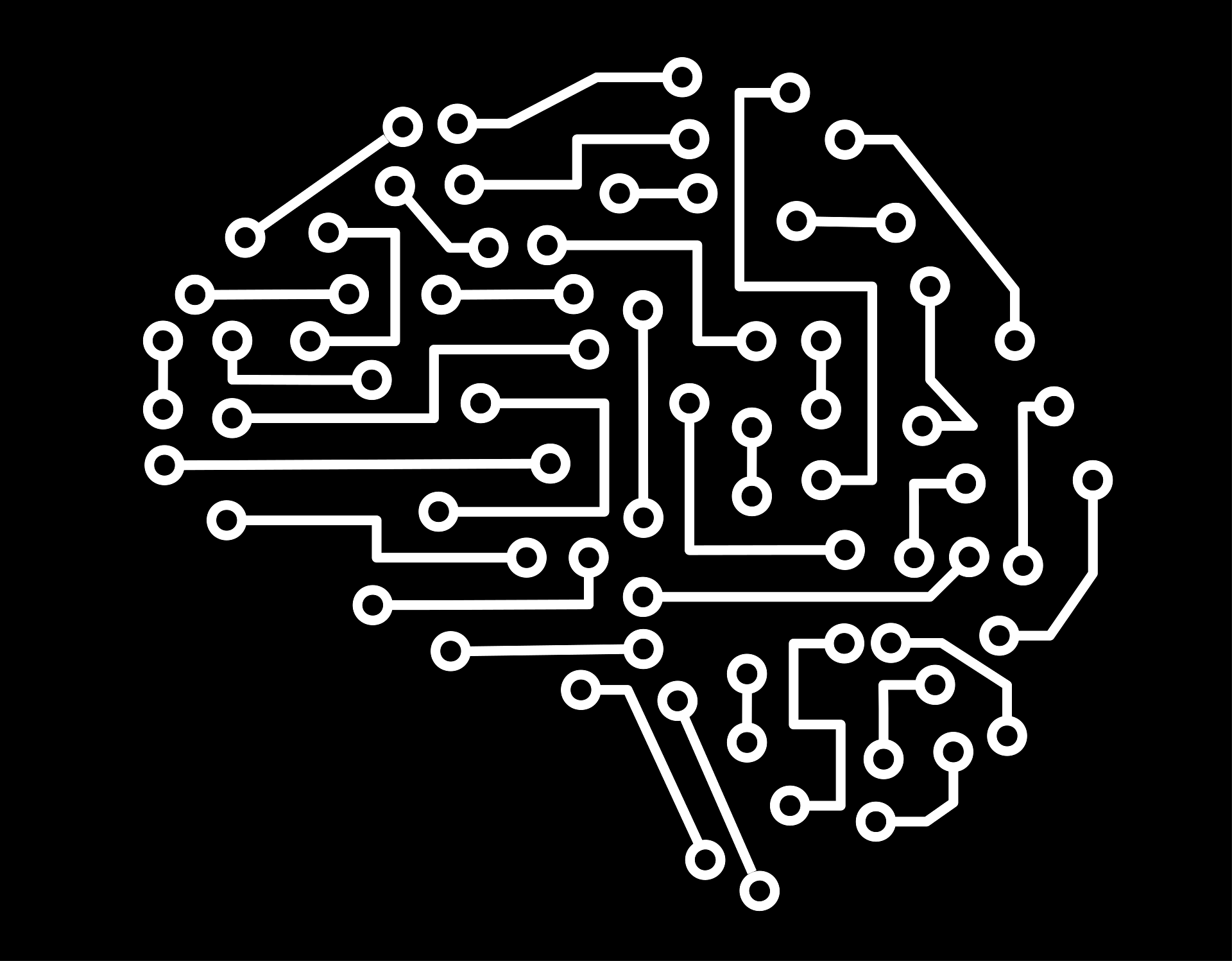
Tackling Data Heterogeneity in Federated Learning
A persistent challenge in Federated Learning (FL) lies in handling statistical heterogeneity—namely, if the clients’ distributions are different from each other. Shinichi Nakajima, BIFOLD research Grouplead and his team propose FLOCO (Federated Learning over Connected Modes), to tackle those issues.
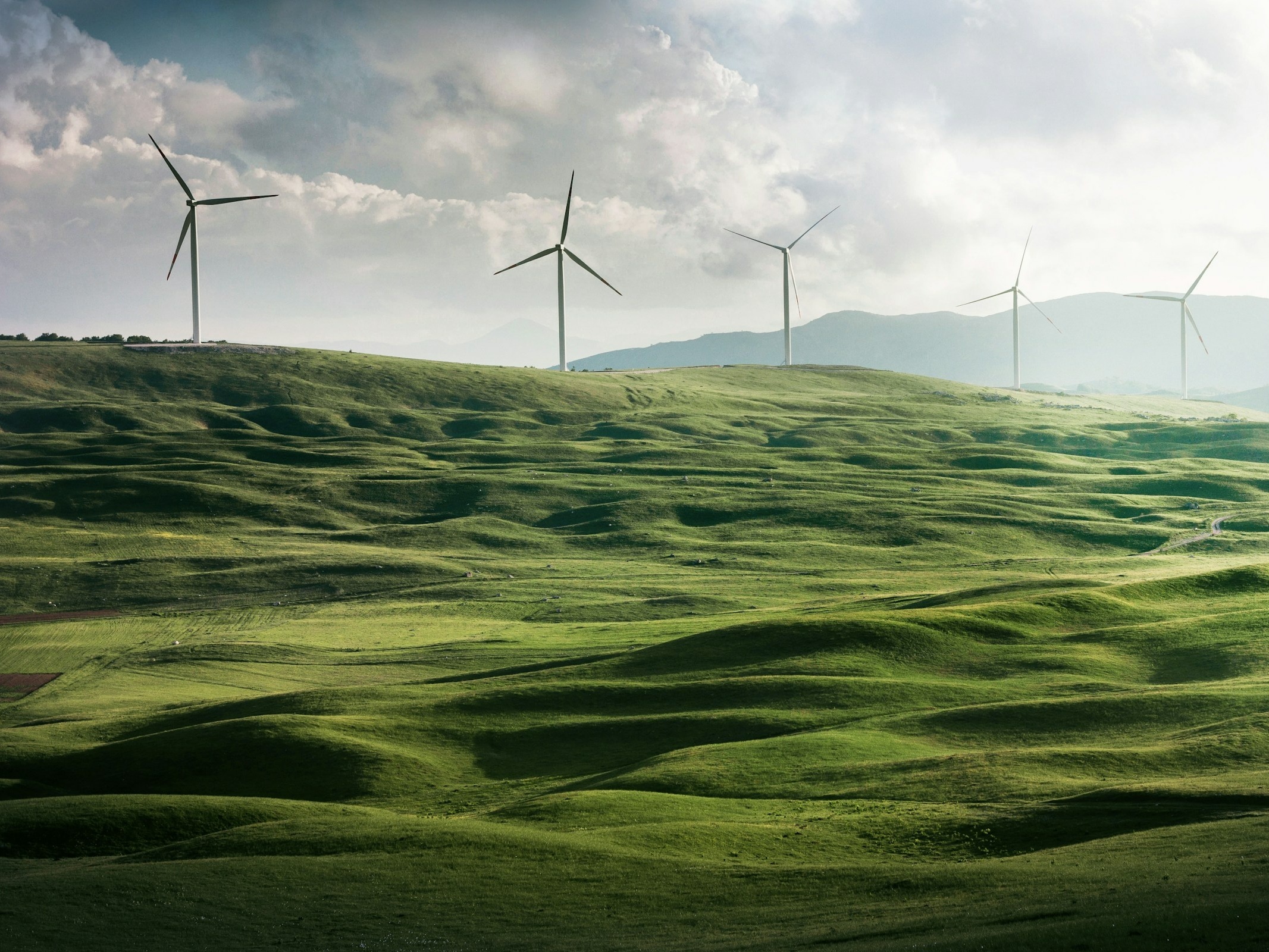
Sustainable Federated Learning
Federated Learning is expected to further increase the power consumption of machine learning, which is already recognized as one of the most energy-intensive computational applications today. However, due to its distributed nature, Federated Learning also offers new opportunities to align this demand with the availability of green energy.