Together with an international team of researchers from Mayo Clinic BIFOLD Co-Director Prof. Dr. Klaus-Robert Müller developed a new type of algorithm to explore which regions of the brain interact with each other. Their results could improve brain stimulation devices to treat disease. For millions of people with epilepsy and movement disorders such as Parkinson’s disease, electrical stimulation of the brain already is widening treatment possibilities. In the future, electrical stimulation may help people with psychiatric illness and direct brain injuries, such as stroke.
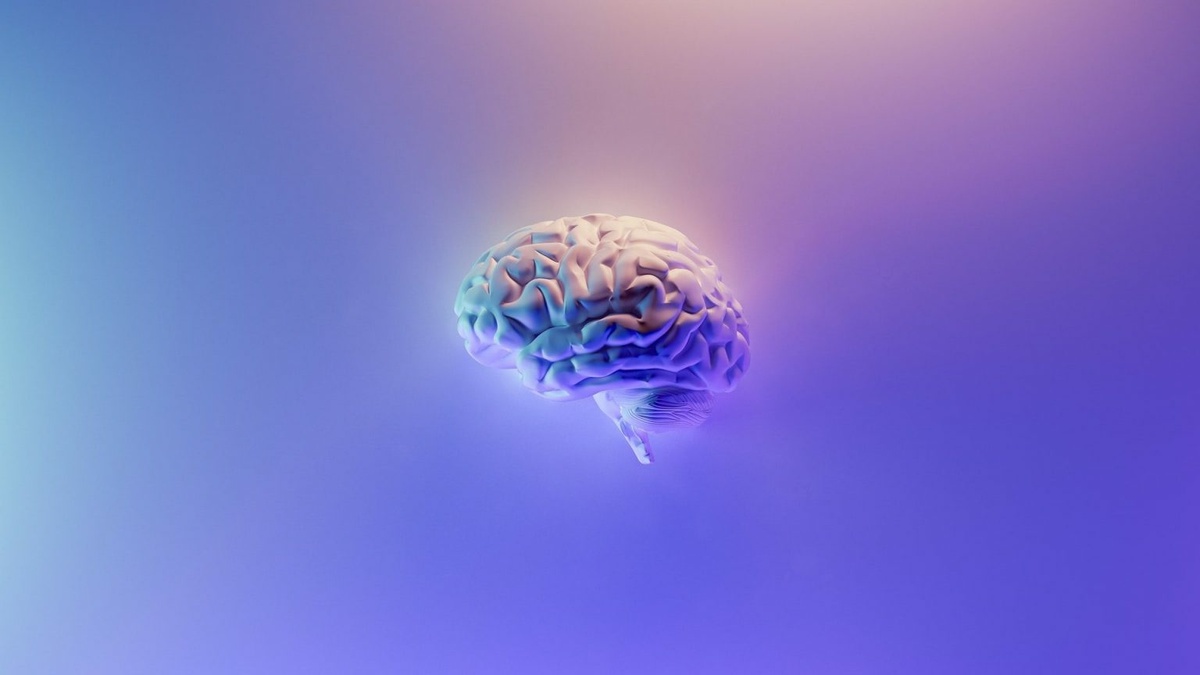
Studying how brain networks interact with each other is complicated. Brain networks can be explored by delivering brief pulses of electrical current in one area of a patient’s brain while measuring voltage responses in other areas. In principle, one should be able to infer the structure of brain networks from these data. However, with real-world data, this is difficult because the recorded signals are complex, and a limited amount of measurements can be made.
To make the problem manageable, the researchers developed a set of paradigms, or viewpoints, that simplify comparisons between effects of electrical stimulation on the brain. Because a mathematical technique to characterize how assemblies of inputs converge in human brain regions did not exist in the scientific literature, the Mayo team collaborated with Klaus-Robert Müller to develop a new type of algorithm called “basis profile curve identification.”
In a study published in PLOS Computational Biology, a patient with a brain tumor underwent placement of an electrocorticographic electrode array to locate seizures and map brain function before a tumor was removed. Every electrode interaction resulted in hundreds to thousands of time points to be studied using the new algorithm.
“Our findings show that this new type of algorithm may help us understand which brain regions directly interact with one another, which in turn may help guide placement of electrodes for stimulating devices to treat network brain diseases,” says Kai Miller, M.D., Ph.D., a Mayo Clinic neurosurgeon and first author of the study. “As new technology emerges, this type of algorithm may help us to better treat patients with epilepsy, movement disorders like Parkinson’s disease, and psychiatric illnesses like obsessive compulsive disorder and depression.”
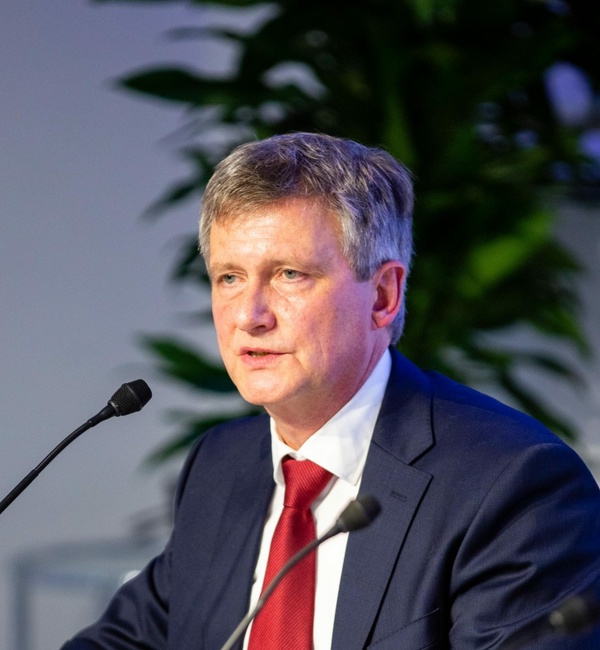
“Neurologic data to date is perhaps the most challenging and exciting data to model for AI researchers,” says Klaus-Robert Müller, study co-author, professor for Machine Learning at Technische Universität Berlin and member of the Google Research Brain Team.”
In the study, the authors provide a downloadable code package so others may explore the technique. “Sharing the developed code is a core part of our efforts to help reproducibility of research,” says Dora Hermes, Ph.D., a Mayo Clinic biomedical engineer and senior author.
This research was supported by National Institutes of Health’s National Center for Advancing Translational Science Clinical and Translational Science Award, National Institute of Mental Health Collaborative Research in Computational Neuroscience, and the Federal Ministry of Education and Research.
The publication in detail:
Kai J. Miller, Klaus-Robert Müller, Dora Hermes: Basis profile curve identification to understand electrical stimulation effects in human brain networks. PLoS Comput. Biol. 17(9) (2021)
>
Brain networks can be explored by delivering brief pulses of electrical current in one area while measuring voltage responses in other areas. We propose a convergent paradigm to study brain dynamics, focusing on a single brain site to observe the average effect of stimulating each of many other brain sites. Viewed in this manner, visually-apparent motifs in the temporal response shape emerge from adjacent stimulation sites. This work constructs and illustrates a data-driven approach to determine characteristic spatiotemporal structure in these response shapes, summarized by a set of unique “basis profile curves” (BPCs). Each BPC may be mapped back to underlying anatomy in a natural way, quantifying projection strength from each stimulation site using simple metrics. Our technique is demonstrated for an array of implanted brain surface electrodes in a human patient. This framework enables straightforward interpretation of single-pulse brain stimulation data, and can be applied generically to explore the diverse milieu of interactions that comprise the connectome.
Brain networks can be explored by delivering brief pulses of electrical current in one area while measuring voltage responses in other areas. We propose a convergent paradigm to study brain dynamics, focusing on a single brain site to observe the average effect of stimulating each of many other brain sites. Viewed in this manner, visually-apparent motifs in the temporal response shape emerge from adjacent stimulation sites. This work constructs and illustrates a data-driven approach to determine characteristic spatiotemporal structure in these response shapes, summarized by a set of unique “basis profile curves” (BPCs). Each BPC may be mapped back to underlying anatomy in a natural way, quantifying projection strength from each stimulation site using simple metrics. Our technique is demonstrated for an array of implanted brain surface electrodes in a human patient. This framework enables straightforward interpretation of single-pulse brain stimulation data, and can be applied generically to explore the diverse milieu of interactions that comprise the connectome.
This article was first published on: newsnetwork.mayoclinic.org