Dr. Marina Höhne, BIFOLD Junior Fellow, researches explainable artificial intelligence funded by the German Federal Ministry of Education and Research.
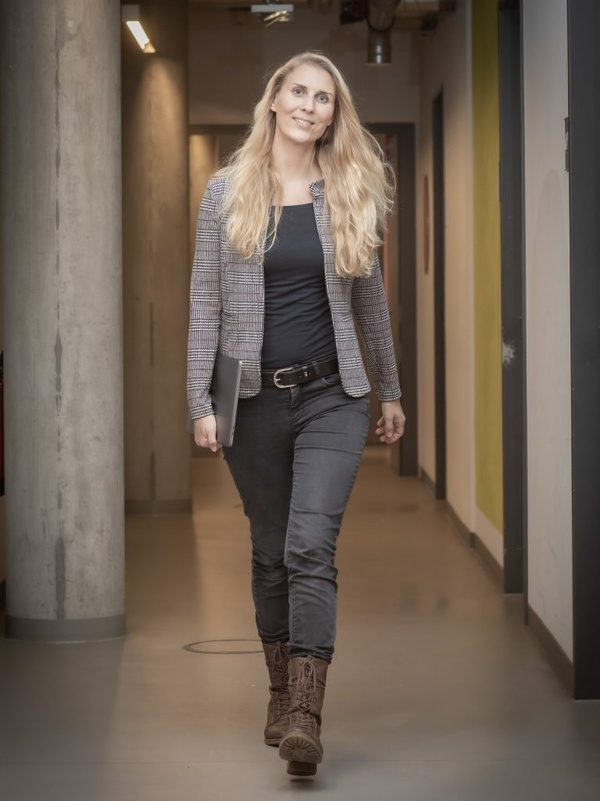
For most people, the words mathematics, physics and programming in a single sentence would be reason enough to discreetly but swiftly change the subject. Not so for Dr. Marina Höhne, postdoctoral researcher at TU Berlin’s Machine Learning Group led by Professor Dr. Klaus-Robert Müller, as well as Junior Fellow at the Berlin Institute for the Foundations of Learning and Data (BIFOLD) and passionate mathematician. Since February 2020, the 34-year-old mother of a four-year-old son has been leading her own research group, Understandable Machine Intelligence (UMI Lab), funded by the Federal Ministry of Education and Research (BMBF).
In 2019, the BMBF published the call “Förderung von KI-Nachwuchswissenschaftlerinnen” which aims at increasing the number of qualified women in AI research in Germany and strengthening the influence of female researchers in this area long-term.
“The timing of the call was not ideal for me, as it came more or less right after one year of parental leave,” Höhne recalls. Nevertheless, she went ahead and submitted a detailed research proposal, which was approved. She was awarded two million euros funding over a period of four years, a sum comparable to a prestigious ERC Consolidator Grant. “For me, this came as an unexpected but wonderful opportunity to gain experience in organizing and leading research.”
A holistic understanding of AI models is needed
The topic of her research is explainable artificial intelligence (XAI). “My team focuses on different aspects of understanding AI models and their decisions. A good example of this is image recognition. Although it is now possible to identify the relevant areas in an image that contribute significantly to an AI system’s decision, i.e. whether the nose or the ear of a dog was influential in the model’s classification of the animal, there is still no single method that conclusively provides a holistic understanding of an AI model’s behavior. However, in order to be able to use AI models reliably in areas such as medicine or autonomous driving, where safety is important, we need transparent models. We need to know how the model behaves before we use it to minimize the risk of misbehavior,” says Marina Höhne outlining her research approach. Among other things, she and her research team developed explainable methods that use so-called Bayesian neural networks to obtain information about the uncertainties of decisions made by an AI system and then present this information in a way that is understandable for humans.
To achieve this, many different AI models are generated, each of which provides decisions based on slightly different parameterizations. All of these models are explained separately and subsequently pooled and displayed in a heat-map. Applied to image recognition, this means that the pixels of an image that contributed significantly to the decision of what it depicts, cat or dog, are strongly marked. The pixels that are only used by some models in reaching their decision, by contrast, are more faintly marked.
“Our findings could prove particularly useful in the area of diagnostics. For example, explanations with a high model certainty could help to identify tissue regions with the highest probability of cancer, speeding up diagnosis. Explanations with high model uncertainty, on the other hand, could be used for AI-based screening applications to reduce the risk of overlooking important information in a diagnostic process,” says Höhne.
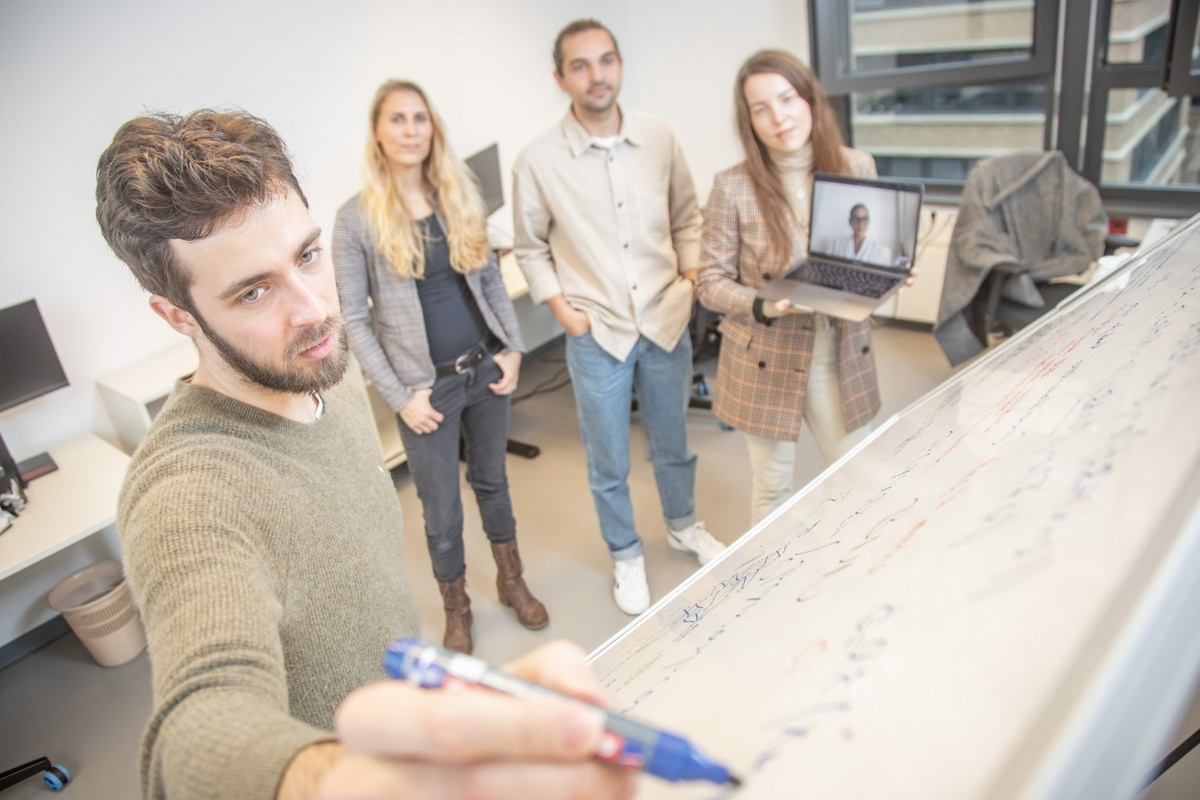
Today, the team consists of three doctoral researchers and four student assistants. Marina Höhne, who in addition is associated professor at the University of Tromsø in Norway, explains that the hiring process of the team came with problems of a very particular nature: “My aim is to develop a diverse and heterogeneous team, partly to address the pronounced gender imbalance in machine learning. My job posting for the three PhD positions received twenty applications, all from men. At first, I was at a loss of what to do. Then I posted the jobs on Twitter to reach out to qualified women candidates. I’m still amazed at the response – around 70,000 people read this tweet and it was retweeted many times, so that in the end I had a diverse and qualified pool of applicants to choose from,” Höhne recalls. She finally appointed two women and one man. Höhne knows all about how difficult it can still be for women to combine career and family. At the time of her doctoral defense, she was nine-months pregnant and recalls: “I had been wrestling for some time with the decision to either take a break or complete my doctorate. In the end, I decided on the latter.” Her decision proved a good one as she completed her doctorate with “summa cum laude” while also increasing her awareness of the issue of gender parity in academia.
Understandable AI combined with exciting applications
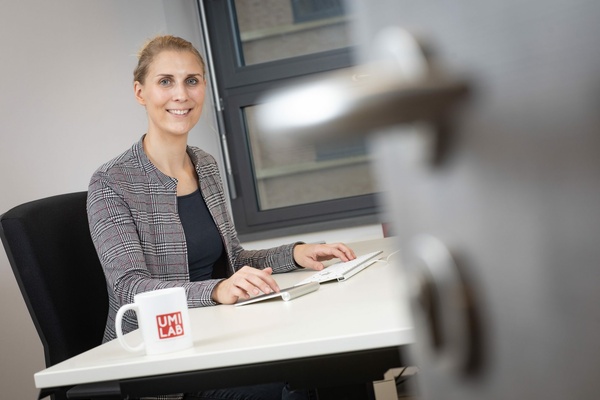
Höhne already knew which path she wanted to pursue at the start of her master’s program in Technomathematics. “I was immediately won over by Klaus-Robert Müller’s lecture on machine learning,” she recalls. She began working in the group as a student assistant during her master’s program, making a seamless transition to her doctorate. “I did my doctorate through an industry cooperation with the Otto Bock company, working first in Vienna for two years and then at TU Berlin. One of the areas I focused on was developing an algorithm to make it easier for prosthesis users to adjust quickly and effectively to motion sequences after each new fitting,” says Höhne. After the enriching experience of working directly with patients, she returned to more foundational research on machine learning at TU Berlin. “Understandable artificial intelligence, combined with exciting applications such as medical diagnostics and climate research – that is my passion. When I am seated in front of my programs and formulas, then it’s like I am in a tunnel – I don’t see or hear anything else.”